In today’s global environment, organizations find themselves vulnerable to ever-more-subtle cyber threats, and simple methods of guarding against cyber threats prove ineffective. As attackers commence using sophisticated methods to avoid identification, the solution to the problem requires rounded approaches to perceive and mitigate threats before they worsen. This is where AI-driven threat hunting comes in handy.
AI-based threat hunting is a modern threat intelligence concept that overlays artificial intelligence (AI), machine learning (ML), and big data analytics to boost threat detection and tracking. Basically, by analyzing large quantities of data, AI can find such patterns, deviations, and threats that analysts can miss. In this blog post, the author will seek to discuss the core elements of threat hunting applied to the AI environment, the pros and cons of the use of threat hunting, different methodological approaches to AI threat hunting, and the prospects of this effective cybersecurity strategy.
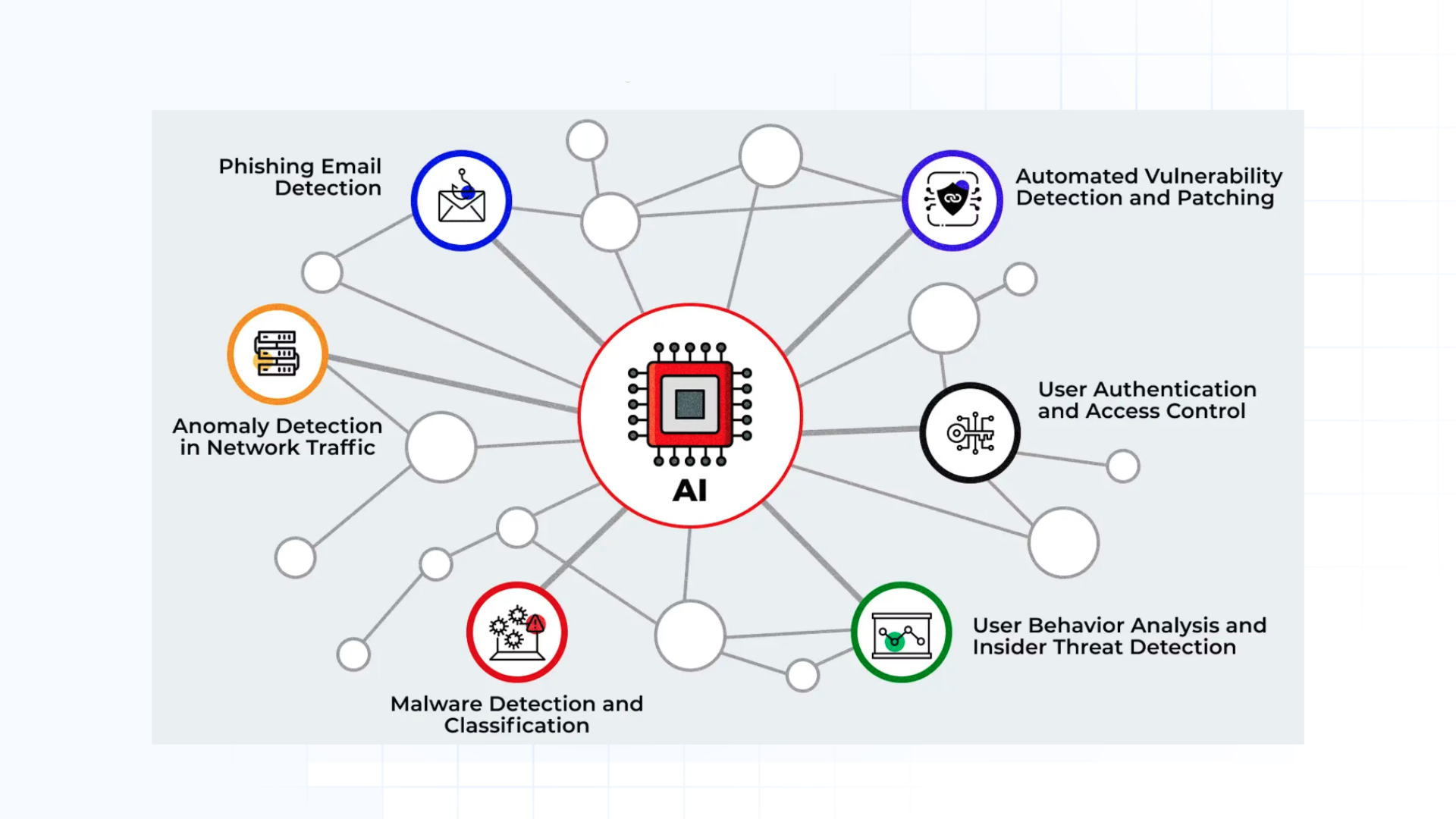
Understanding Threat Hunting
Threat hunting, also known as threat intelligence, is the process of searching for threats on a network before they become realized. In contrast to conventional, largely automated approaches to detecting security threats, threat hunting exploits human judgment.
Threat Hunting as an Effective Solution for Active Defense
As cyberattacks become more sophisticated, organizations face numerous challenges, including:
- Increased Attack Surface: As organizations embark on the digital transformation journey by migrating to the cloud and IoT and embracing remote working, their attack surfaces are growing.
- Advanced Persistent Threats (APTs): APTs are slow and persistent hacking activities that focus on certain targets and often stay hidden for long periods before the target information leakage is discovered.
- Reactive Security Measures: Traditional security solutions aim to detect security threats after an incident, making an organization a sitting duck for new threats.
AI and Machine learning handle these challenges well in AI-driven threat hunting, which improves threat detection and response.
Threat Hunting and the Part Played by AI
AI is at the epicentre of shifting threat hunting from a responsive to a proactive landscape. Here are some key aspects of how AI enhances threat hunting:
- Data Analysis at Scale: Computer-based threat hunting can analyze information from network connections, endpoint events, and numerous threat feeds. This capability enables security teams to examine trends or structures and determine whether something is wrong in their organization.
- Predictive Analytics: Statistical methods can be learned from previous instances to identify future threats. By examining previous attacks and their features, AI can consequently find IOCs and create models that will allow the detection of similar threats in real-time.
- Automated Investigation: AI can help ease threat-hunting tasks by assisting with the first steps of the process. Automated investigations can encompass data connection, context augmentation, and simple threat profiling and help analysts prioritize more complex tasks involving the application of human reasoning.
- Continuous Learning: By the nature of artificial intelligence, systems can learn from new data and threats that are constantly emerging. As new attack techniques and methods emerge, the machine can adjust its models and enhance its ability to detect threats that organizations face.
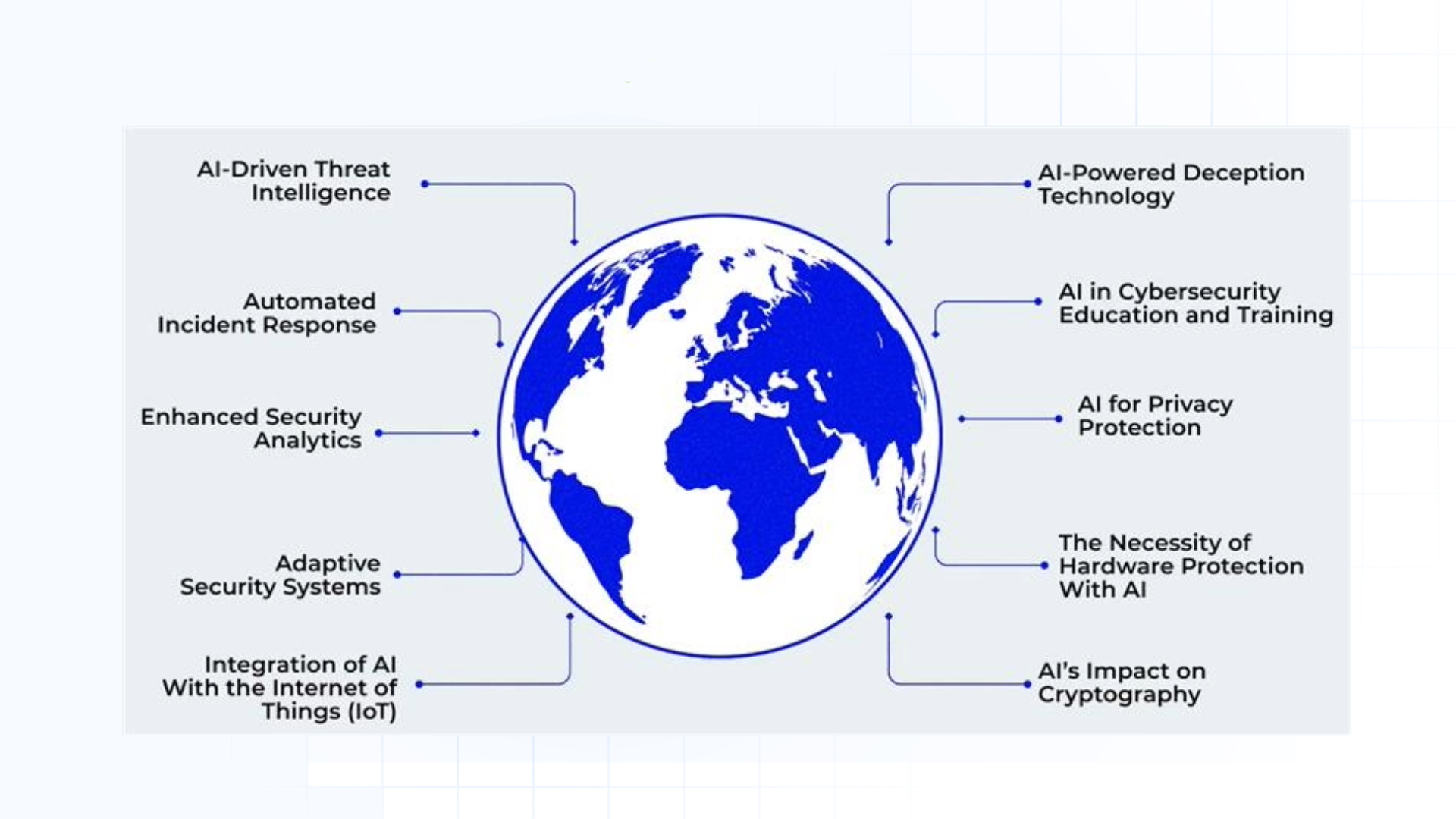
Advantages of AI-sponsored threat hunting
Implementing AI-driven threat hunting provides organizations with several advantages:
- More Threat Identification and Mitigation: AI can help save time spent on threat identification and reaction. It can identify odd patterns in the data stream necessary for incident detection and response.
- Enhanced Accuracy: AI technology threat hunting reduces the generation of false positives owing to patterns and contextual analysis. This results in more accurate threat identification, instead of receiving a large number of notifications and having to spend a lot of time identifying what are real threats.
- Improved Resource Allocation: AI delivers threat-hunting capabilities that involve pre-setting common procedures so that security teams can free up their time and switch it to other important work. The analysts can, therefore, spend most of their time on higher analyses like strategic planning and enhanced investigations rather than a lot of time on analysis.
- Scalability: Artificial intelligence technologies can handle ever-growing data volumes as an organization expands. This scalability also ensures that security measures penetrate every other network layer without necessarily extruding a signal.
- Proactive Defense: By identifying risky situations based on various indicators, organizations can build a proactive defence strategy. This proactive approach allows the teams to take preventive measures against these weaknesses in case they are used against the organization.
Threat Hunting using AI-based techniques
There are several methodologies that organizations can adopt to implement AI-driven threat hunting effectively:
- Threat Intelligence Processing: This integration allows the enhancement of threat intelligence feeds within the context of threat hunting. This includes information on identified threats, risks, and adversaries’ processes/techniques that are most useful during hunting.
- Behavioural Analysis: AI can also compare users’ and entities’ behaviours to check if they perform bizarrely. This behavioural analysis allows for determining the insider threat, compromised accounts, and other manifestations of the breach.
- Anomaly Detection: According to machine learning concepts, organizations can easily identify irregularities in the patterns of network communication, application working frequency, and users’ activities. It is most effective in revealing threats often unseen by conventional fixed signature methods.
- Automation of Hunting Tasks: Integration and employment of the automation process relieves time, which requires spending on data collection and processing, for more productive tasks such as attempts to minimize the concept of bad threats. It can mean entering scripts to request the data be typed or following playbooks throughout an investigation.
- Operation and Administration of knowledge: The thinking culture is also motivated by the ability to discuss and share information concerning threats in the cyber world and threats in general with threat hunters, incident responders and IT teams. This cooperation enhances the overall effectiveness of risk searching with artificial intelligence techniques and also enhances processes.
Issues of AI-based threat hunting
While AI-driven threat hunting offers numerous benefits, organizations must also navigate several challenges:
- Data Quality and Availability: An AI-driven threat hunt mainly depends on data, its quality, and availability. If this information is incorrect or incomplete, then all the hunting efforts may return false positives or miss potential threats.
- Skills Gap: The cybersecurity industry desperately needs skilled professionals, and organizations struggle to source employees with the right skill sets to drive AI threat-hunting solutions.
- Complexity of Implementation: The adoption of AI in security processes is even more challenging than the application of technologies as it may require a reinvention of the specific procedure to complement the use of AI. This means that organizational systems and processes must be avowed and aligned to support AI technologies and applications and/or that an organization possesses requisite infrastructural support.
- Evolving Threat Landscape: This remains so because threat actors are not static; they constantly invent new ways to avoid detection. Moreover, AI-driven systems should be able to learn from newly acquired data to remain effective against new threats.
- Concerns of ethical and privacy point of view: Adopting threat hunting based on AI technologies increases ethical and privacy problems, such as collecting and monitoring. Organs must deal with some issues to avoid losing users’ trust and follow the rules.
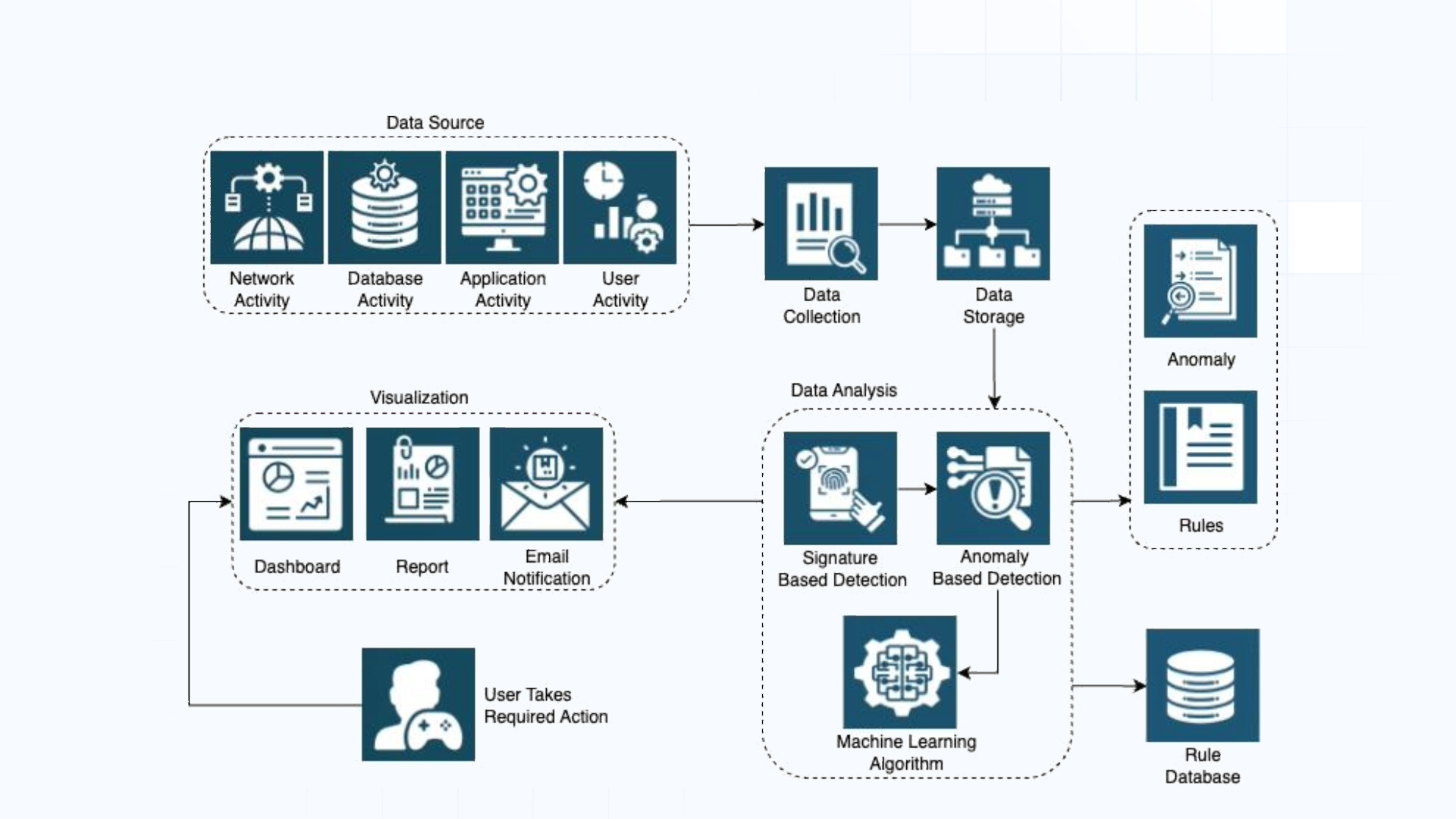
Towards the future of AI-driven threat hunting
The future of AI-driven threat hunting looks promising, with several key trends expected to shape its evolution:
- Increased Automation: The threat-hunting process will continue to be automated as AI technologies develop with time in organizations. This automation will help security teams better realize threats and respond faster.
- Enhanced Collaboration: AI systems will also integrate more closely with human analysts, which means that teams could rely on the strengths of both. AI will analyse the collected data and routine work, while humans will uncover crucial investigations and conduct profound decision-making.
- Integration with the Security Operation: Modern threat hunting will be powered by artificial intelligence and become an inseparable component of security operations, strengthening organizations’ responses to threats and increasing the effectiveness of security as an overall process.
- Proactive Threat Hunting: This change was already underway and will continue as business leaders pursue threat hunting in anticipation of threats.
- More Importance to Data Security: With emerging doubts about data fidelity, organizations must be sensitive to ethical issues as they deploy AI-driven threat-hunting solutions. The challenges include complying with regulations and retaining users’ trust.
Final Thoughts
The use of AI in threat hunting is a definite step up in the overall functionality of cybersecurity. AI and machine learning help organizations improve threat identification and response and reduce the overall risk of an organization. Since cyber threats are only going to get worse, implementing passive tools like AI threat hunting will be a necessity for corporations that want to safeguard their reputation and goods. This is where threat hunting with the help of AI comes into play; despite numerous challenges, the value proposition of AI-based threat hunting is reasonably obvious, and organizations that invest in those technologies will be in a relatively favourable position regarding future cybersecurity.