Overview
The combination of computer vision and healthcare is revolutionizing the way we diagnose and plan treatment. Thanks to AI-powered tools, especially in analyzing medical images, we are seeing remarkable improvements in how we diagnose cancer and diseases and deliver quality care to patients.
This blog will examine the latest computer identification techniques for biomedical images, focusing on how they help in tasks such as breaking down and classifying medical images and connecting them to electronic health records (EHRs).
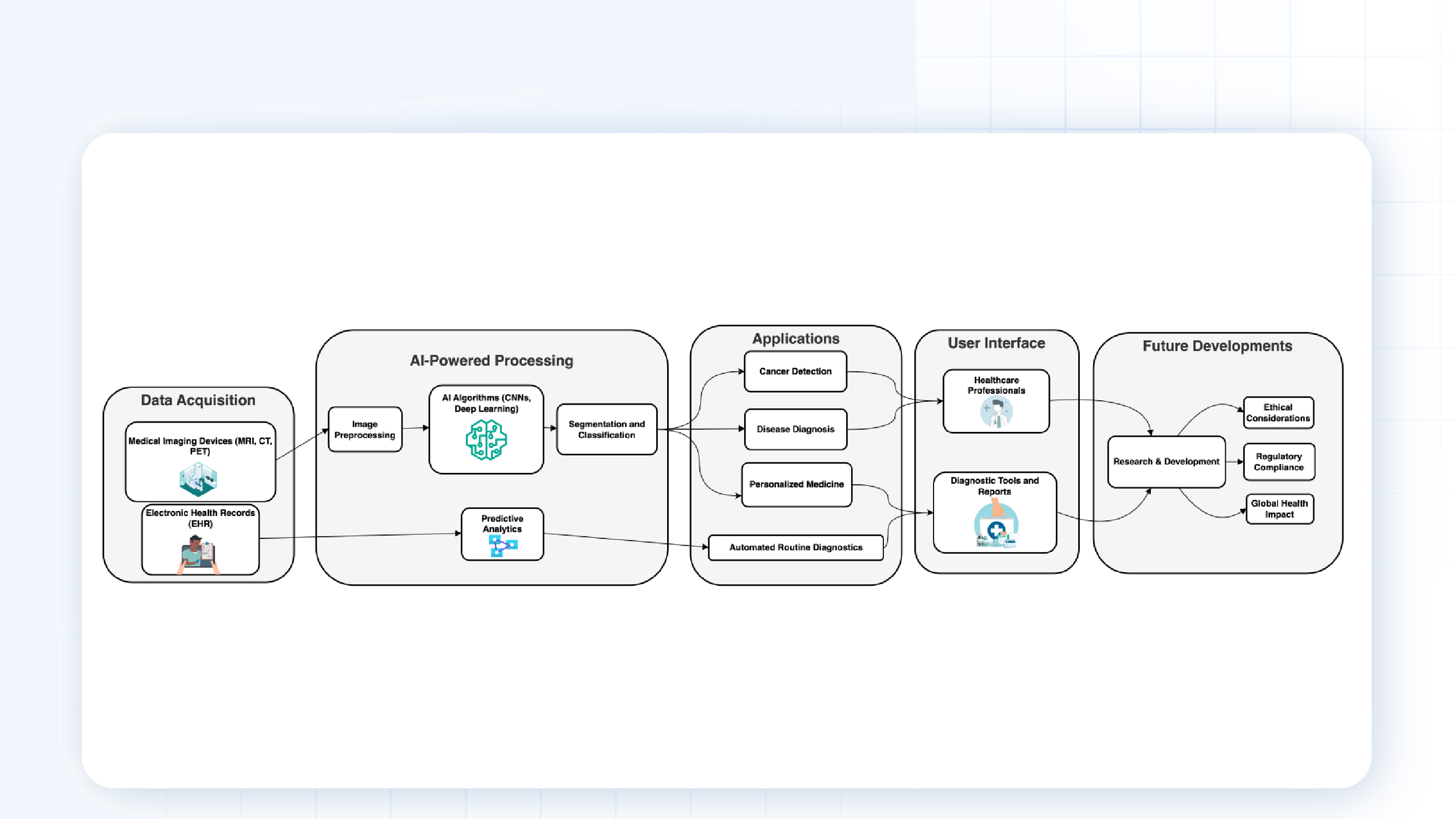
Computer Vision’s Impact on Healthcare
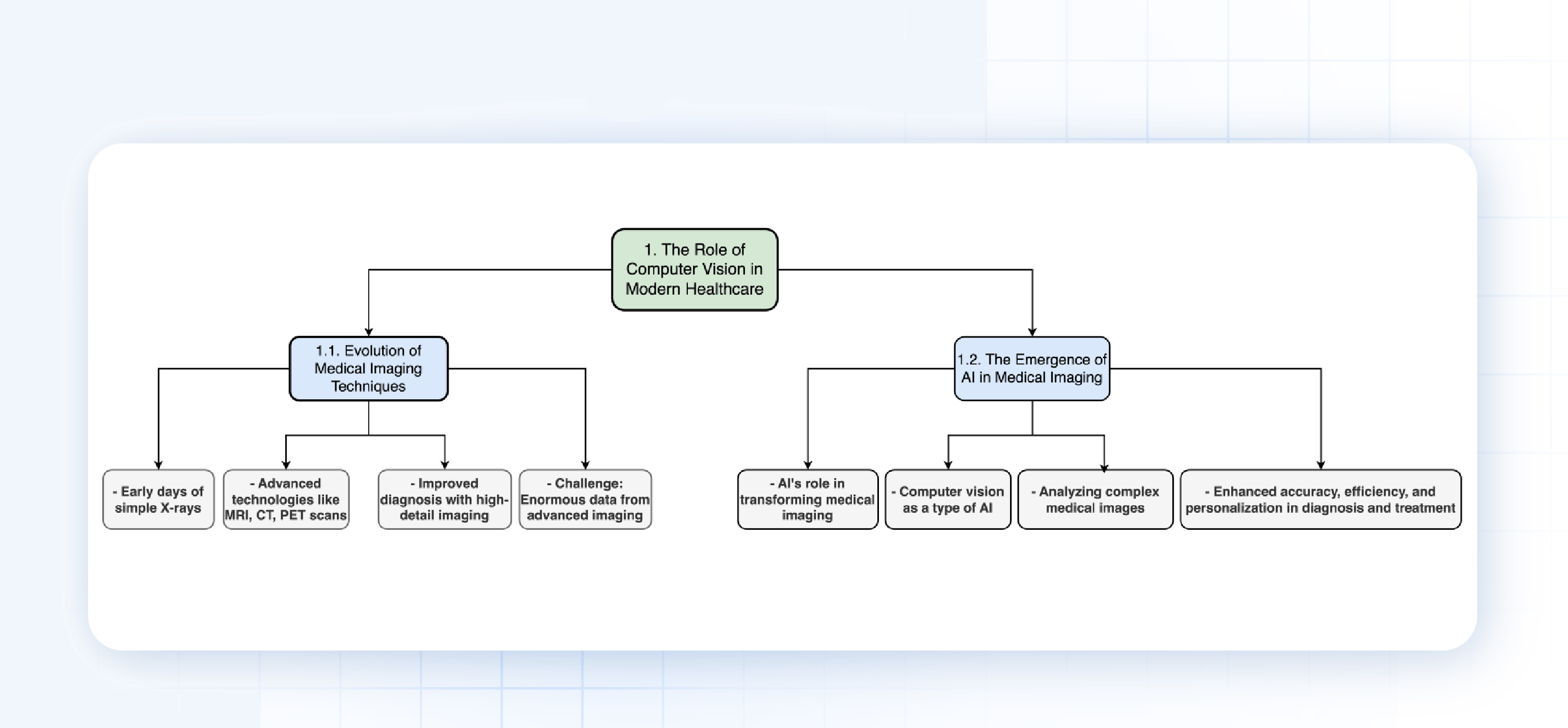
Evolution of Medical Imaging Techniques
Medical imaging has come a long way since the first simple X-rays. Today, we have advanced technologies such as MRI (Magnetic Resonance Imaging), CT (Computed Tomography), and PET (Positron Emission Tomography) that provide incredibly detailed views of the human body. These advances have improved our ability to accurately diagnose conditions. The downside, however, is that these sophisticated imaging techniques generate a large amount of data, which can be difficult to analyze quickly and accurately
The Emergence of AI in Medical Imaging
This is where Artificial Intelligence (AI) comes into play. AI, especially in computer vision, is changing Medical Imaging. Computer vision is a form of AI that allows machines to understand and interpret visual information from the world around them. In healthcare, computer vision is being pursued to analyze complex medical images with accuracy and speed beyond human capabilities. Integrating computer vision into medical imaging systems is transforming how we diagnose and treat disease, making the entire process more accurate, efficient, and personalized.
Applications in Cancer Detection and Disease Diagnosis
Improving Early Cancer Detection
Breast Cancer: Transforming Mammography
Breast cancer is one of the most common cancers affecting women worldwide, and early detection is essential to improve survival. Mammography has long been a commonly used screening tool but often relies heavily on the expertise and judgment of radiologists. This can sometimes lead to differences in interpretation, as different radiologists may see or emphasize different things on the same mammogram. Furthermore, early signs of breast cancer, such as microcalcifications, are often very subtle and can easily be missed.
This is where computer vision systems have a big impact. By analyzing mammograms with incredible accuracy, these AIs can detect microcalcifications, which are small calcium deposits in breast tissue that could indicate the beginning of cancer, for example, which can be an early sign of breast cancer. These patterns can be so simple that even experienced radiologists won’t notice them, but the AI can always capture them, ensuring that no potential signs of cancer are overlooked.
In addition to identifying these early signatures, computer vision algorithms can also help reduce false positives, where non-cancerous conditions can be mistaken for cancer Not that this keeps patients from getting it stress and procedures that are not only necessary, but also help focus medical resources on what is most needed. Overall, AI-driven advances in breast screening provide more accurate and earlier diagnosis, which is crucial for successful treatment and better outcomes
Lung Cancer: Accurate Analysis of CT scans
Lung cancer is one of the most deadly cancers, often diagnosed late when treatment options are limited and prognosis is poor. Early lung cancer may have no obvious symptoms, and even when symptoms do appear, they are easily dismissed as non-severe conditions such as infection or chronic obstructive pulmonary disease (COPD). This makes early detection by imaging even more important.
CT scanning is a powerful tool for early detection of pulmonary nodules that may be indicative of lung cancer. However, correct interpretation of these scans is difficult because not all nodules are cancerous. Some are benign or from diseases other than cancer. Traditional methods of CT image interpretation can sometimes struggle to distinguish between such nodules, resulting in false positives or missed diagnoses.
Computer vision is changing this aspect of lung cancer diagnosis. AI algorithms can analyze CT scans in detail to distinguish between benign and malignant nodules with more accuracy than traditional methods. These algorithms have been trained on large datasets of medical images to differentiate subtle differences in nodule size, shape, and texture that may indicate cancer.
These AI tools accurately diagnose lung cancer, increasing the chances of early, treatable diagnosis. This early detection is very important, as it increases the chances of proper treatment being highly effective and can save lives. Moreover, these tools can reduce the number of biopsies and unnecessary surgeries by providing more reliable information on potentially cancerous and non-cancerous tissues.
Expanding Disease Diagnosis Beyond Cancer
Eye Health: Detecting Diabetic Retinopathy
Diabetic retinopathy is a serious complication of diabetes that affects the eyes. It happens when high blood sugar levels damage the blood vessels in the retina, which is responsible for detecting light and transmitting signals to the brain. If left untreated, diabetic retinopathy can lead to vision loss or even blindness. The condition usually goes on without symptoms until it gets worse, so early detection is important to prevent irreversible vision loss.
AI-powered computer vision systems now play an important role in the early stages of diabetic retinopathy. These tools analyze detailed images of the retina, looking for small changes that may indicate the onset of the disease, such as small blood vessels, microaneurysms, and other abnormalities in blood vessels, or these changes can be more subtle and lost to vision in routine inspection, especially if testing is done without special equipment.
A key advantage of this AI technology is its ability to bring high diagnostic capabilities to remote or underserved areas In many parts of the world, basic eye care is not available, and those with diabetes do not have their eyes checked regularly. A.I. This allows for early detection and treatment to begin before it progresses, thus preventing more blindness.
Heart Health: AI in Echocardiography
Cardiovascular diseases (CVDs) are a leading cause of death worldwide, and early detection is key to the effective management and treatment of these conditions. As ultrasound waves are used to image the heart, echocardiography is more of a diagnostic tool for heart disease. This allows doctors to see how the heart is working, assess its structure and function, and spot any abnormalities that could indicate heart disease.
However, interpretation of echocardiograms can be complex and requires a high level of expertise. Images produced by echocardiography can vary depending on angle, equipment type, and patient condition, complicating accurate diagnosis. Echocardiograms are now interpreted using AI-driven computer vision systems. These algorithms can classify and analyze parts of the heart, such as chambers, valves, and blood vessels. By doing so, they can measure cardiac function such as ejection fraction (the percentage of blood pumped out of the heart per beat) and cardiac defects that can detect abnormalities such as valve failure dysfunction, cardiomyopathy (diseases of the heart muscle), and inherited.
The main advantage of using AI in echocardiography is the ability to precisely the assessment process, reduce variability, and increase accuracy. This is particularly important in clinical situations where rapid and reliable assessment is required to guide treatment decisions. In addition, A.I.
In summary, the application of computer vision in disease diagnosis extends far beyond cancer, offering significant benefits in the early detection and management of other serious conditions such as diabetic retinopathy and cardiovascular diseases. By improving the accuracy, accessibility, and efficiency of diagnostic processes, AI-driven computer vision is helping to transform healthcare and improve patient outcomes across a wide range of diseases.
AI for Medical Image Segmentation
Advanced Medical Image Segmentation
Accurate segmentation of medical images is a critical component of modern healthcare, particularly in the treatment of complex conditions like cancer. Medical image segmentation involves dividing an image into meaningful sections, such as isolating a tumor from surrounding tissues. This process is essential for precise treatment planning, as it allows doctors to target affected areas while sparing healthy tissue.
- Tumor Segmentation: Precision in Oncology: Tumor segmentation is especially important in cancer treatment. Computer vision algorithms, powered by techniques like convolutional neural networks (CNNs), are now capable of automatically identifying and outlining the boundaries of tumors in imaging studies such as MRIs or PET scans. This precision is crucial for planning surgeries, radiation treatments, and other interventions. By accurately distinguishing between cancerous and healthy tissues, these AI tools ensure that treatments are as effective and minimally invasive as possible.
- Multi-Organ Segmentation: Complex Case Management: When dealing with cases like metastatic cancer, where multiple organs may be involved, treatment planning becomes significantly more complex. AI-driven segmentation tools can simultaneously segment different organs and tissues, allowing for a comprehensive view of the affected areas. This capability is vital for ensuring that all cancerous tissues are targeted during treatment while minimizing damage to healthy organs. The result is a more tailored and effective treatment plan, even in the most complicated cases.
Sophisticated Image Classification Techniques
Classifying medical images is another area where AI is making a profound impact. Classification involves categorizing segments of an image to determine the nature of what is being observed, such as distinguishing between benign and malignant tissues. This step is fundamental to diagnosis and subsequent treatment planning.
- Machine Learning in Image Classification: Machine learning, particularly through deep learning models like CNNs, has transformed the field of medical image classification. These models can be trained on large datasets of labeled medical images, enabling them to classify new images with a high degree of accuracy. For example, in dermatology, AI models can analyze images of skin lesions and classify them as either benign or malignant, often with accuracy comparable to that of expert dermatologists. This not only speeds up the diagnostic process but also makes it more accessible, particularly in regions where specialist care is not readily available.
Computer Vision and EHR Integration
Enhancing Personalized Medicine
- Cross-Referencing Imaging Data with Patient Histories: The integration of computer vision with Electronic Health Records (EHRs) is a major step forward in personalized medicine. By combining imaging data with detailed patient histories — including genetic information and past treatments — AI can deliver more precise and individualized diagnostic insights. For example, when an AI system analyzes a patient’s MRI scan, it can also consider the patient’s entire medical history, helping to provide a diagnosis and suggest treatment options that are uniquely tailored to that individual’s health profile.
- Predictive Analytics for Disease Progression – When AI-driven computer vision systems are integrated with EHRs, they can also be used for predictive analytics, which involves identifying patterns and predicting how a disease might progress. This is specifically useful for managing chronic conditions like diabetes or cardiovascular diseases. By monitoring and analyzing imaging data over time, AI can spot early signs of complications or worsening conditions, allowing for timely adjustments in treatment to prevent serious outcomes.
Streamlining Clinical Workflows
- Automation of Routine Diagnostics: Bringing together computer vision and EHRs enables the automation of routine diagnostic tasks, reducing the time and effort required from healthcare professionals. AI can automatically analyze imaging studies and highlight potential issues, freeing up clinicians to focus on more complex cases and decisions. This automation makes clinical workflows more efficient, leading to quicker diagnoses and better use of medical resources.
- Improving Data Accuracy and Interoperability: One common challenge with EHRs is ensuring data accuracy and smooth interoperability between different systems. AI-driven tools can help by standardizing imaging data and ensuring it is correctly linked to the relevant patient records. This standardization allows healthcare providers to access accurate and up-to-date information easily, improving the coordination of care and decreasing the chances of medical errors.
Future Trends in Healthcare Computer Vision
Ongoing Research and Development
The field of computer vision in healthcare is evolving rapidly, with continuous research aimed at enhancing the accuracy, efficiency, and reach of AI-driven tools. Innovations like unsupervised learning and more advanced neural network architectures are expected to further expand computer vision’s capabilities in analyzing biomedical images.
Ethical and Regulatory Considerations
As AI becomes more important in healthcare, there is a growing need for clear ethical guidelines and strong regulatory frameworks. Ensuring that AI algorithms are transparent, fair, and secure is essential to earning the trust of healthcare professionals and patients alike. Regulatory bodies are increasingly focusing on the approval and oversight of AI-driven medical devices and software, making sure they meet strict safety and efficacy standards.
Potential Impact on Global Health
The potential global impact of AI-driven computer vision in healthcare is immense. By making advanced diagnostic tools more accessible, especially in areas with limited resources, these technologies can help close the healthcare gap, providing high-quality care to populations that have historically been underserved.
Conclusion: Transforming Healthcare
The integration of computer vision into Biomedical Image Analysis and Diagnostics opens a new era in precision medicine. From improving early detection of cancer to enabling personalized treatment plans, AI-powered technology is transforming healthcare. As this technology continues to evolve and become more integrated into healthcare systems, including EHRs, the potential for better patient outcomes and more efficient healthcare becomes more apparent.
The future of healthcare will be characterized by continuous improvement and increased adoption of advanced computer vision features. This will lead to a world where medical diagnosis is faster, more accurate, and more tailored to each patient’s individual needs. This is just the beginning of a journey that is random with endless possibilities.