The revolution of genomics research today is artificial intelligence (AI), which is revolutionizing data analysis, discovery, and clinical genomics applications. Among these driver technologies are advancing genome analysis and phenotyping, focusing on rare diseases and cancer, using AI-powered computer vision.
AI uses machine learning, deep learning, and multi‐modal data processing to learn how to understand the underlying biological processes that play out in diseases and, at the same time, improve diagnostic accuracy. This blog briefly examines the transformative power of AI and computer vision in genome analysis, its application in life science, and what remains to be done.
How Gen AI is Revolutionizing All Industries?
Gen AI, as the name implies, is generative for industries, offering value additions in efficiency, precision, and capacity. However, its function in healthcare is more innovative — researchers and clinicians can use it to solve many problems. In genomic medicine, therefore, AI is opening possibilities in areas such as disease diagnosis, drug development, and individualized therapy.
What is Genomic Analysis?
Genomic analysis means the study of or comparison of a whole organism’s set of DNA and the genes that comprise it. This involves the identification of genetic differences, the analysis of gene activity, and the recognition of factors that may cause health and illness. This analysis is critical in the diagnosis of genetic diseases, the identification of their aetiology, and the resultant development of treatment therapies.
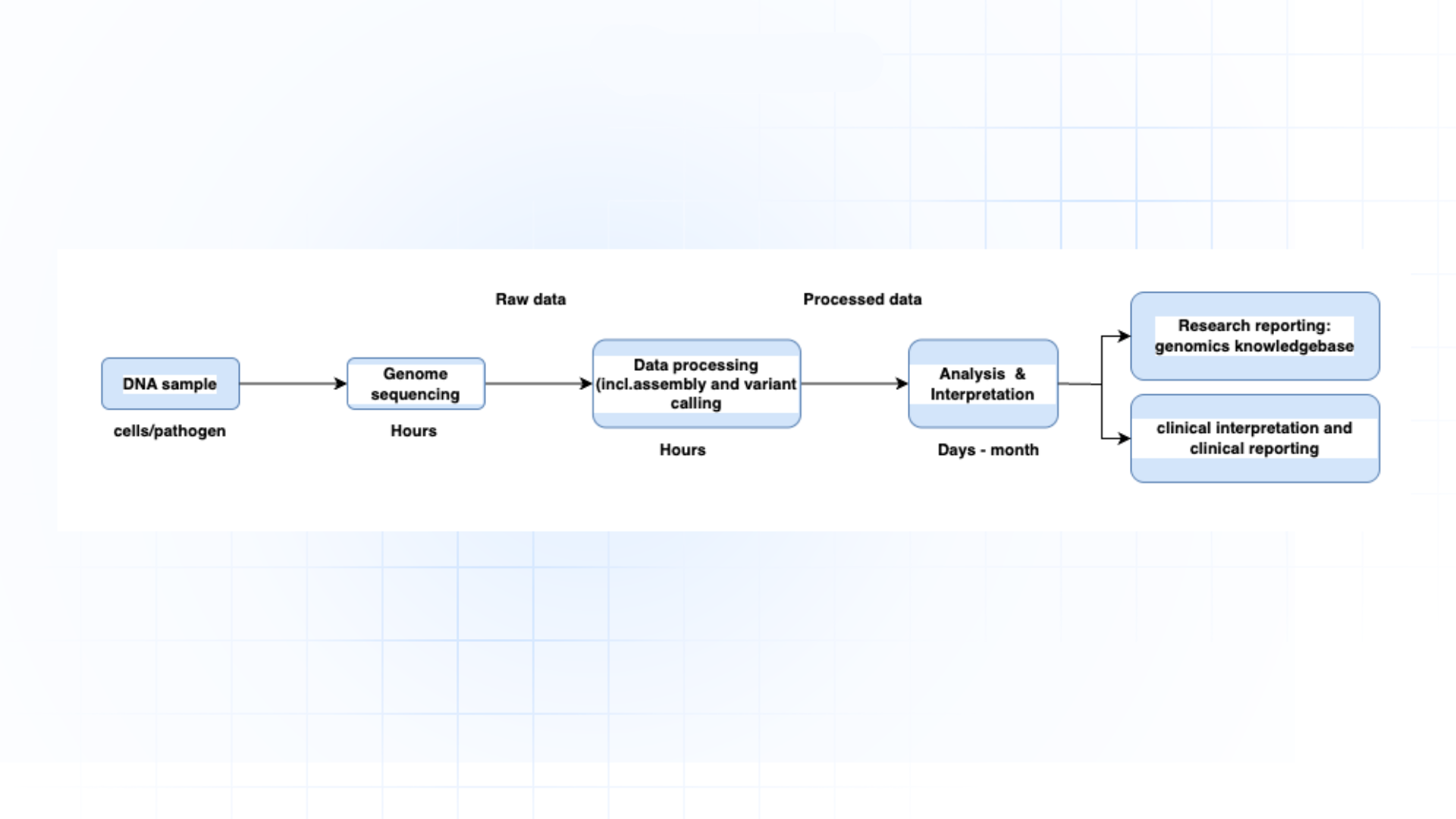
This can help to determine the functions of genes and regulatory sequences and their involvement in genetic disorders. The result is providing the key for molecular individual and targeted therapy.
Requirement of AI & Computer Vision in Genome Analysis
The nature and amount of genomic data call for sophisticated computational technologies. AI and computer vision are valuable as they can process ‘big data’, diminish human errors, and find patterns and features without taking time in lengthy, repetitive processes.
These technologies are essential for:
- Phenotyping: Determining some physical characteristics that are associated with genetic differences.
- Variant Analysis involves Selecting and ranking clinically significant variants. Multi-omics integration involves integrating genomic data with other molecular data types to provide a holistic approach to diseases.
- Genome Editing: Improving both accuracy and reliability of the current genetic editing techniques.
AI & Computer Vision in Genome Analysis
AI and Computer Vision are revolutionizing how genomic data is captured and interpreted in the genomics science sector. Machine learning and deep learning enable high-throughput data analysis for pattern recognition while utilizing integrated gene ontology to predict gene-disease association.
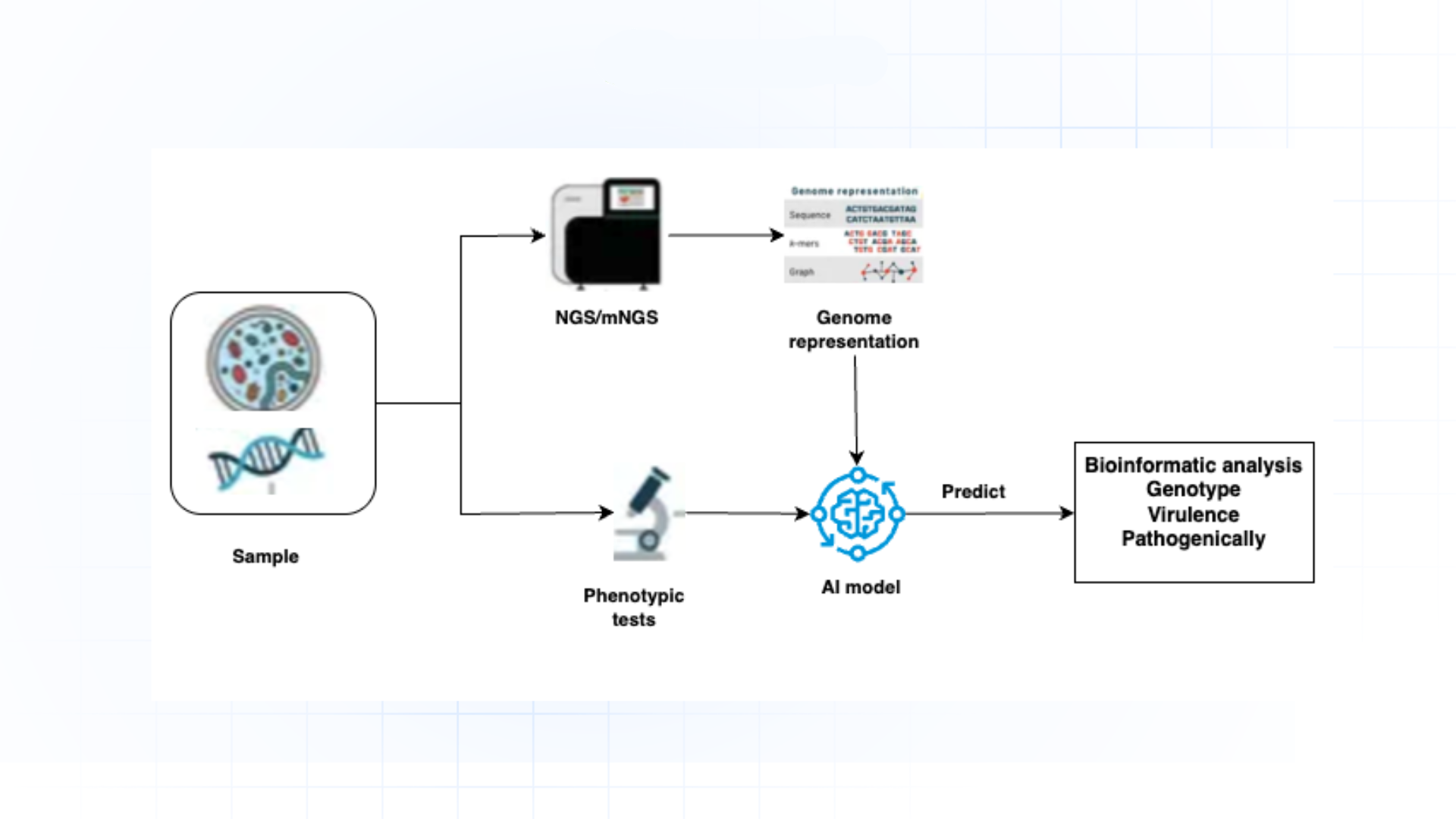
Computer vision in this process enhances the standard operation of reviewing genetic images, such as chromosomal karyotypes or histology slides, to check for abnormalities. As one set of reviewed authors stated, ‘These technologies will help to streamline the layers of analysis and make the workflow real-time, as well as help reproducibility of genomic research.
Automating Genomic Data Analysis
Automation in genomic analysis is critical for improving efficiency and accuracy. AI algorithms can perform tasks like variant filtering, annotation, and ranking, enabling faster and more reliable diagnostics.
- Rapid Diagnosis in ICU Settings: Rapid diagnosis of genetic disorders can be lifesaving in neonatal intensive care units (ICUs). Researchers developed an AI pipeline incorporating natural language processing (NLP) to extract phenotypic data from electronic health records (EHRs).
- The system achieved 97% sensitivity and 99% precision in diagnosing genetic diseases among critically ill infants. This approach demonstrates the potential of AI to streamline genomic diagnostics in clinical settings, although adaptation to diverse hospital systems remains a challenge.
AI and Computer Vision in Genomic Phenotyping
Due to polygenic structure, phenotyping — the analysis of external characteristics and their links to genetic and environmental factors — is fundamental for understanding multifactorial diseases. Decentralized computing approaches, such as AI computer vision algorithms, are shedding light on this sector.
For instance, many single/offbeat genetic disorders are expressed as some distinct facial features or craniofacial anomalies. Machine learning algorithms fed by big phenotype-genotype databases can analyse face images and offer possible genotype syndromes in this analysis.
Advancing Cancer Genomics Through AI
Cancer genomics has been revolutionized by combining machine learning, genomics, and imaging. Computer vision methods aid digital pathology processes and enhance tissue-level image assessment efficiency. For instance, headline features such as histology image analysis have been incorporated with genomic data to improve the predictive accuracy of cancer prognosis.
Gene expression profiles and imaging data that include source features in large amounts typically constitute high-dimensional datasets. These high-dimensional data structures can be fed into deep learning models and advanced computational clusters to identify ‘hidden’ structures and interaction values. This capability enhances the identification of biomarkers and therapeutic objectives for different kinds of cancer Real-Time Intelligence.
Multi-Omics Integration: A Holistic View of Genomics
Because genomic data is commonly analyzed in combination with other ‘omics data, it is crucial to mention the combination of genomics with transcriptomics, proteomics, and metabolomics. The materials have outlined that the multi-modal approach offers enough knowledge about the diseases’ nature and patient variations.
The application of AI methodology, Deep Learning, is growing as a tool for analysing high-dimensional multi-attribute genomics datasets, resulting in new relationships between genetic and cellular dynamics.
Use Cases of Genome Analysis
Face2Gene
- Problem: Identifying subtle facial patterns associated with rare genetic syndromes often requires expert analysis, which is time-consuming and unpredictable.
- Solution Approach: Face2Gene, developed by FDNA, leverages the DeepGestalt algorithm, a deep-learning model trained on thousands of patient cases. The platform analyzes facial images to suggest potential genetic syndromes based on visual patterns. This approach automates and accelerates the diagnosis of rare genetic conditions, assisting clinicians in making more accurate and faster decisions in Data Science.
Key Benefits
- Achieves 90% accuracy in predicting syndromes within the top ten suggestions.
- Assists clinical and laboratory geneticists in diagnosing rare genetic syndromes.
- Reduces the time and variability in expert-based diagnoses.
- Improves diagnostic efficiency for complex genetic conditions.
AI in Histology Image Analysis
- Problem: Manual analysis of histological images is labour-intensive and limited in scalability.
- Solution Approach: AI-driven deep learning models process histological images, integrating them with genomic data to enhance cancer diagnosis. These models help identify biomarkers and predict cancer progression, providing previously difficult insights to uncover manually. Automating image analysis improves both scalability and diagnostic accuracy.
Key Benefits
- Accelerates biomarker discovery for cancer diagnostics.
- Enhances diagnostic accuracy in cancer detection.
- Integrates genomic data to improve prognosis predictions.
- Increases scalability in histology image analysis.
- Reduces the labour-intensive nature of the manual histological analysis.
Diagnosing Lower Respiratory Tract Infections (LRTIs)
- Problem: Diagnosing LRTIs in critically ill patients requires integrating complex multi-omics data.
- Solution Approach: Machine learning models process multi-omics data, including RNA and DNA sequence data from pathogens, microbiomes, and host responses, to diagnose LRTIs in critically ill patients. The system can make precise diagnoses by integrating complex datasets, offering a powerful tool for clinicians dealing with such infections.
Key Benefits
- Enhances clinical diagnostics in critically ill patients.
- Achieves accurate diagnosis by integrating multi-omics data.
- It helps identify pathogens and host responses more effectively.
- Improves the potential for personalized treatments.
- Demonstrates the value of integrating complex biological data for accurate diagnosis.
AI in CRISPR Gene Editing
- Problem: Predicting off-target effects and ensuring the reliability of gene-editing protocols.
- Solution Approach: AI algorithms analyze CRISPR gene-editing protocols to predict off-target effects and enhance the precision of gene-editing processes. These models assess potential risks and ensure gene edits’ accuracy, improving gene therapies’ safety and efficacy.
Key Benefits
- Improves the safety of CRISPR-based gene therapies.
- Minimizes off-target effects, ensuring precise gene editing.
- Increases the reliability of gene-editing protocols.
- Facilitates the development of more effective therapeutic interventions.
- Accelerates advancements in genomic medicine by refining gene-editing techniques.
Benefits
AI-powered computer vision in genomics offers several advantages:
- Efficiency: Evaluation saves much time and effort needed when analyzing the data.
- Accuracy: Machine learning helps refine the analysis of variant implications and, consequently, the patients’ phenotyping.
- Scalability: AI, in particular, assists in handling and interpreting large data sets, which would otherwise be challenging when conducting research at such a large scale.
- Personalization: Specific knowledge obtained through the generally used AI-assisted components helped apply the appropriate therapeutic style.
Challenges in AI-Driven Genomics
- Data Quality and Accessibility: High-quality annotated data sets are crucial for creating reliable AI models. Nonetheless, large-scale DNA data can differ significantly in quality or content across different institutions.
- Regulatory and Ethical Concerns: AI’s application in clinical genomics raises questions about regulatory evidence measures, validation criteria, and the ethics of autochthonous decision-making.
- Integration with Clinical Workflows: Translation from research to practice remains a major challenge. A genome pipeline, for instance, needs to be integrated seamlessly into various healthcare environments and health IT systems, and this must not compromise throughput precision or yield.
Future Directions for Genome Analysis
- Expanded Multi-Omics Analysis: Including additional categories of ‘omics data with clinical and environmental data will improve disease understanding on multiple levels.
- Personalized Medicine: AI models will assist in developing therapies that consider the relationship between a patient’s specific genetic markers, phenotypes, and health records.
- Real-Time Genomics: Progress in automation and computing will allow the analysis of large amounts of genomic data in real-time, thus extending the application of precision medicine.
- Open-Source Collaboration: The use of open-source tools will only continue to increase, expanding the future of AI technologies in genomics.
- Interpretability of AI Models: This calls for improved explainability of AI models to gain clinical practitioners’ trust.
Conclusion
Deep learning and biologically inspired imaging have extended genomics’ applicability into phenotyping pipelines, variant calling, and multi-home integration. Face2Gene, as well as various automated analysis pipelines and applications of AI in genome editing, are examples of the great potential of these technologies to change clinical and research fields.
However, addressing challenges like data quality, ethical issues, and clinical implementation will be crucial to realise AI’s full potential in genomic medicine. As the abovementioned obstacles are addressed, the application of AI in genomics is expected to represent an ever-increasing focus within the field of Life Science and Personalized Medicine.