Introduction
Artificial intelligence (AI) and sports analytics have significantly changed how teams handle game strategy, player development, and scouting. Researchers and analysts use advanced statistical methods, machine learning algorithms, and computer vision techniques to uncover previously unavailable insights.
Football started using sports analytics a bit later than other sports. Still, there are already several innovative approaches from various parts of AI, like statistical learning (SL), computer vision (CV), and game theory (GT), to help team managers, coaches, and players make better decisions. For instance, simple statistical learning tools can now categorize player types, predict team trajectories, or evaluate the Impact of a player’s actions, like passes or tackles, leading to a goal. Game theory, which looks at decision-making when multiple players are involved, helps understand players’ strategies during moments like penalty kicks and predict their actions. Recent advances in computer vision are being used to track players, analyze their postures, and even predict injuries by looking at things like how they walk and their level of tiredness.
Statistical Learning (SL) in Football
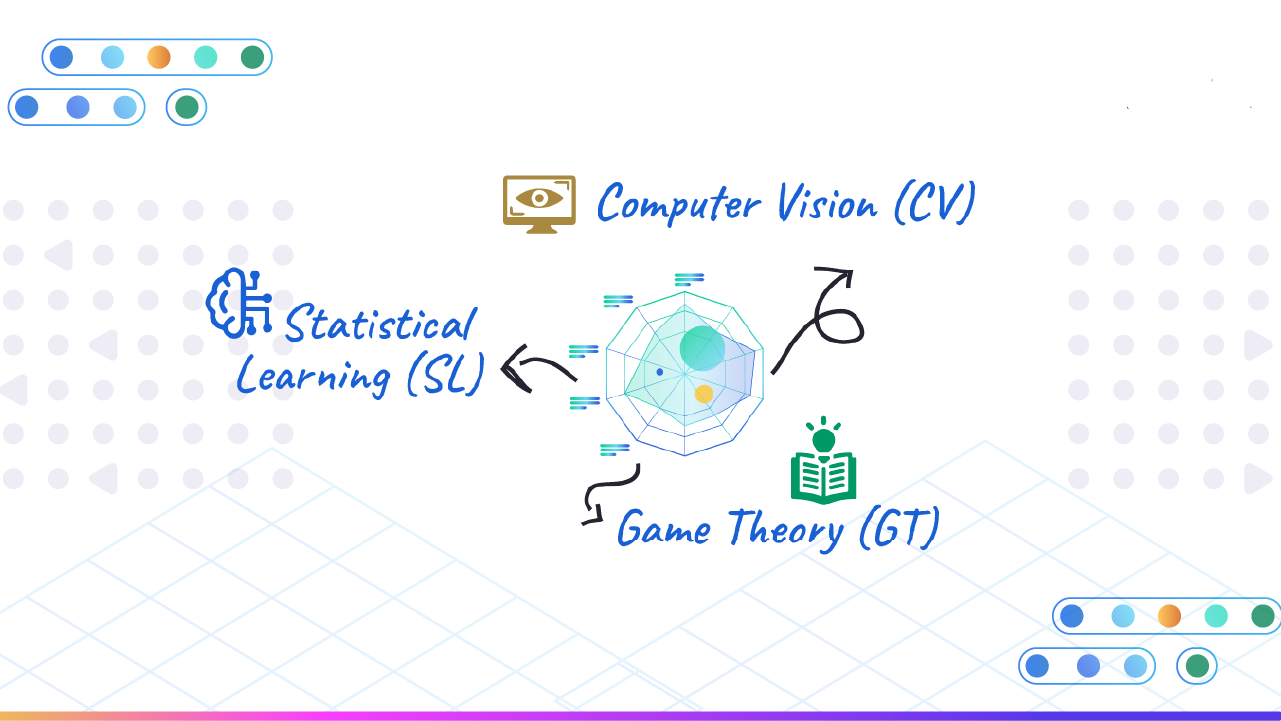
Predictive Modeling of Player Performance: When a football team wants to sign a new striker, they can use statistical learning to build a predictive model. This model is based on various player metrics collected from previous seasons, such as goals per match, shots on target, and passing accuracy. By analyzing this data, the team’s analysts can predict how well the player will perform in the future and assess whether they fit the team’s tactical needs. For example, the team might find that a particular striker excels in converting chances when playing against high-pressing defenses. This aligns with the team’s counter-attacking style and could make the player a good fit for the team.
Game Theory (GT) in Football
Analysis of Penalty Kicks: Game theory can help analyze the penalty kicks by modelling the strategic choices of the penalty taker and goalkeeper. In this game, the taker can shoot left, right, or centre, while the goalkeeper can dive in any direction or stay in the centre. Analysts can use mixed-strategy Nash equilibrium to predict the optimal strategies for both players. This helps to improve the team’s penalty-taking and defending tactics.
Computer Vision (CV) in Football
Automatic Highlight Generation: Automated Highlight Generation is a technology that uses computer vision to create match highlights without human input. By teaching machine learning models on copious amounts of video data, these systems can learn to identify critical events in a football match, such as goals, saves, and significant changes. For instance, a model might analyze the ball’s movement, the players’ positions, and the crowd’s reaction to detect when a goal has been scored. This technology streamlines the production of highlight reels and helps analysts quickly review crucial moments of the game.
Challenges in Analysis by Statistical Learning
Analyzing Football through statistical learning involves tackling several unique and complex challenges, given the sport’s dynamic nature and the several factors influencing game outcomes. Here are detailed explanations of these challenges with examples to illustrate the points:
1. Characterizing Playing Styles: Quantifying playing styles involves translating the abstract qualities of teams and players into measurable statistics. For instance, a team known for its aggressive pressing style could be characterized by metrics such as the number of high intensities runs, tackles in the opponent’s half, and recoveries in advanced positions. Similarly, a player’s dribbling ability could be quantified by successful take-Ons, dribbles per game, and the ability to maintain possession under pressure.
2. Evaluating Impact on the Pitch: Assessing a player’s or team’s impact involves more than goal contributions. For example, a midfielder might control the game’s tempo, contributing to the team’s dominance by making key passes that do not directly result in goals but maintain possession and create pressure. Analyzing such contributions requires looking at pass completion rates, the number of key passes, and the player’s positioning and movement during critical phases of the game.
3. Temporal and Counterfactual Predictions: Predicting future actions in Football is inherently uncertain due to the sport’s unpredictability. For instance, forecasting a player’s movement to receive a pass involves considering several factors, such as the player’s speed, the opposition’s defensive setup, and the passing player’s skill. Statistical models need to account for these variables to make accurate predictions.
4. Credit Assignment for Actions: In a sport where goals are scarce, assigning credit to actions that lead to scoring opportunities is challenging. For example, the defender’s initial tackle that regained possession might be as crucial as the final assist, even though it does not directly result in a goal. Advanced models are required to evaluate the significance of such actions within the game’s broader context.
5. Complex Game States Analysis: Understanding the value of an action, like a pass, requires analyzing the entire game state, which includes the positioning of all players, their potential actions, and the overall game context. An effective pass in Football is not just about the ball reaching another player but also about how it affects the team’s tactical setup and creates opportunities.
6. Leveraging Unsupervised Learning: Due to Football’s complex nature, applying unsupervised learning to discover complex characteristics and playing styles from raw data presents a significant challenge. For instance, identifying a new, statistically substantial playing style involves analyzing vast amounts of game data to find recurring patterns without predefined categories.
7. Temporal Credit Assignment: Assigning credit for actions in Football is complicated by the extended nature of play sequences. For example, a sequence leading to a goal might start with the goalkeeper’s save, followed by a series of passes before the final shot. Determining the contribution of each action requires models that can analyze the entire sequence in the context of the game’s flow.
8. Bridging Statistical and Human Analysis: Integrating statistical findings with the qualitative assessments of coaches and analysts is a significant challenge. For instance, a statistical model might identify a player’s high Impact based on various performance metrics. However, translating these findings into actionable insights that resonate with coaching strategies requires a deep understanding of football tactics and methodologies.
By addressing these challenges, football analytics can advance significantly, offering more profound insights into the game and enabling teams to optimize strategies, player development, and performance analysis.
Challenges in applying Game Theory to sports
Applying game theory to sports presents several challenges, reflecting sports competitions’ complex and dynamic nature and their strategic interactions. Here are some of the key challenges:
1. Complexity of Strategic Interactions: Sports involve multiple stakeholders (players, teams, coaches, management, and fans) with varying objectives and strategies. Capturing all strategies and outcomes in a game-theoretic model can be extraordinarily complex.
2. Human Behavior and Irrationality: Game theory often assumes rational behaviour, but athletes and teams may only sometimes act rationally. Emotional, psychological, and social factors can significantly influence decision-making in ways that traditional game theory models might not accurately capture.
3. Incomplete Information: Unlike many theoretical games, sports competitions often require more complete information. Teams and players may not fully know their opponents’ strategies, conditions, or intentions, making applying game theory models that assume complete information challenging.
4. Dynamic Nature of Sports: Sports conditions and strategies constantly change. Injuries, player development, technological advancements, and weather conditions can alter the strategic landscape, making static game theory models less applicable.
5. Quantifying Payoffs: In game theory, outcomes are often represented by payoffs, which can be challenging to quantify in sports. While winning is a clear objective, other factors like player development, team cohesion, and long-term strategic goals are more complex to measure but equally important.
6. Multiplicity of Equilibria: Sports can have multiple equilibrium outcomes, making it difficult to predict the outcome of strategic interactions. Various equilibria can complicate the analysis and application of game theory to sports.
7. Adaptation and Learning: Teams and players adapt and learn over time, adjusting their strategies based on past experiences. This dynamic learning process can make applying static game theory models problematic, as these models may need to account for adaptive behaviors.
8. Ethical and Governance Considerations: The application of game theory to sports must also consider ethical issues and governance rules. Strategies that might be optimal from a game-theoretic perspective could violate sportsmanship, ethics, or specific regulations of the sport.
Addressing these challenges requires a nuanced understanding of game theory and the specific sports being analyzed. Advanced modelling techniques, including agent-based modelling and the incorporation of behavioral economics, can help overcome some of these challenges, making the application of game theory to sports more accurate and relevant.
Challenges in applying computer vision to football analytics
Applying computer vision to football analytics presents several challenges. These challenges can range from technical to environmental and addressing them is crucial for effectively using computer vision in this domain. Here are some of the critical issues:
1. Variability in Lighting and Weather Conditions: Football games are played in diverse lighting conditions, including varying times of day and weather conditions. This variability can significantly affect the performance of computer vision algorithms, which may struggle to consistently recognize patterns, players, or the ball under different lighting or weather scenarios.
2. Occlusions: Players frequently obstruct each other on the field, making it difficult for computer vision systems to track individual players, the ball, or specific actions. This occlusion issue complicates player tracking, pose estimation, and action recognition.
3. Camera Angles and Quality: The angle and quality of the video feed can significantly impact the effectiveness of computer vision applications. Wide shots may need to provide more detail for accurate analysis, while close-ups can limit the field of view. Furthermore, the video feed resolution and frame rate can limit visual analytics’ accuracy.
4. Complex Player Movements and Interactions: Football is a dynamic sport with complex player movements and interactions. Accurately capturing and interpreting these movements and interactions requires sophisticated models that can handle the complexity and variability of the game.
5. Real-Time Processing Requirements: Analytics often need to be processed in real-time or near-real-time to be helpful during a game. Given the computational complexity of many computer vision tasks, this poses a significant challenge.
6. Data Annotation and Model Training: Developing accurate computer vision models for football analytics requires substantial amounts of annotated data. Collecting and labelling this data is time-consuming and expensive. Moreover, the models must be continuously updated with new data to remain accurate.
7. Integration with Existing Analytical Tools: Integrating computer vision-based insights with football analytics tools and workflows can be challenging. It requires technical integration and ensuring the insights are actionable and meaningful to coaches, analysts, and other stakeholders.
Overcoming these challenges requires ongoing research and development in computer vision, machine learning, and domain-specific knowledge of Football. Technology advances and collaboration between technologists and football professionals are vital to unlocking the full potential of computer vision in football analytics.
Integration of statistical learning, game theory, and computer vision
As AI research areas have independently shown their value in football analytics, the real potential lies in combining statistical learning, computer vision, and game theory. This combination could lead to a more integrated approach, like an Automated Video Assistant Coach (AVAC), which would enhance the game for players, coaches, and fans by improving understanding and performance in Football.
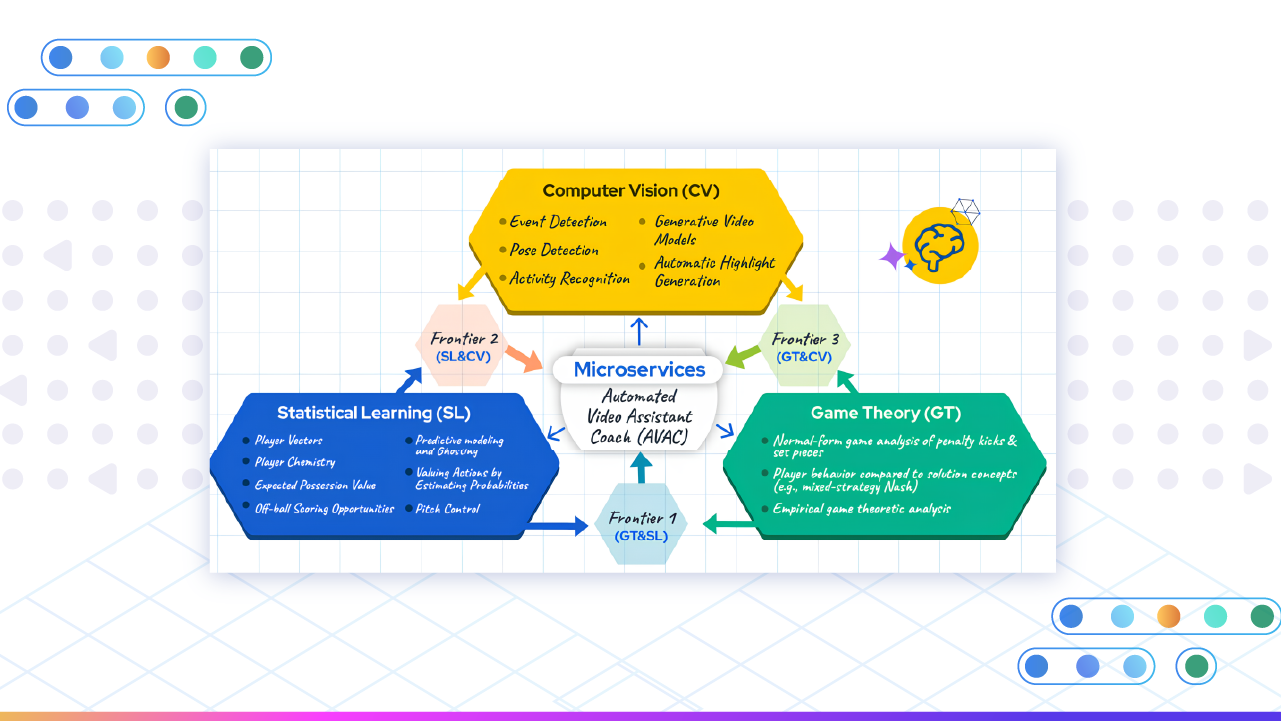
Integration of statistical learning, computer vision, and game theory
Improving football analytics involves learning representations of individual players and identifying an effective means of contextualizing or ranking entire teams. Traditional team ranking methods often need to pay more attention to teams nuanced, long-term playing styles and capture the dynamics of individual games. To address these challenges, there are two primary goals:
- (i) learning representations that accurately characterize the long-term playing styles of football teams and
- (ii) developing contextual representations of teams that can effectively depict in-game dynamics.
The integration of predictive and prescriptive models with game-theoretic analysis is anticipated to be closely aligned, fostering a constructive interaction between this research direction and the identified frontiers of Game Theory and Computer Vision (GT&CV) and Game Theory and Statistical Learning (GT&SL). This interdisciplinary combination promises new opportunities for coaches and decision-makers, such as predictive models focusing on football players at the trajectory level.
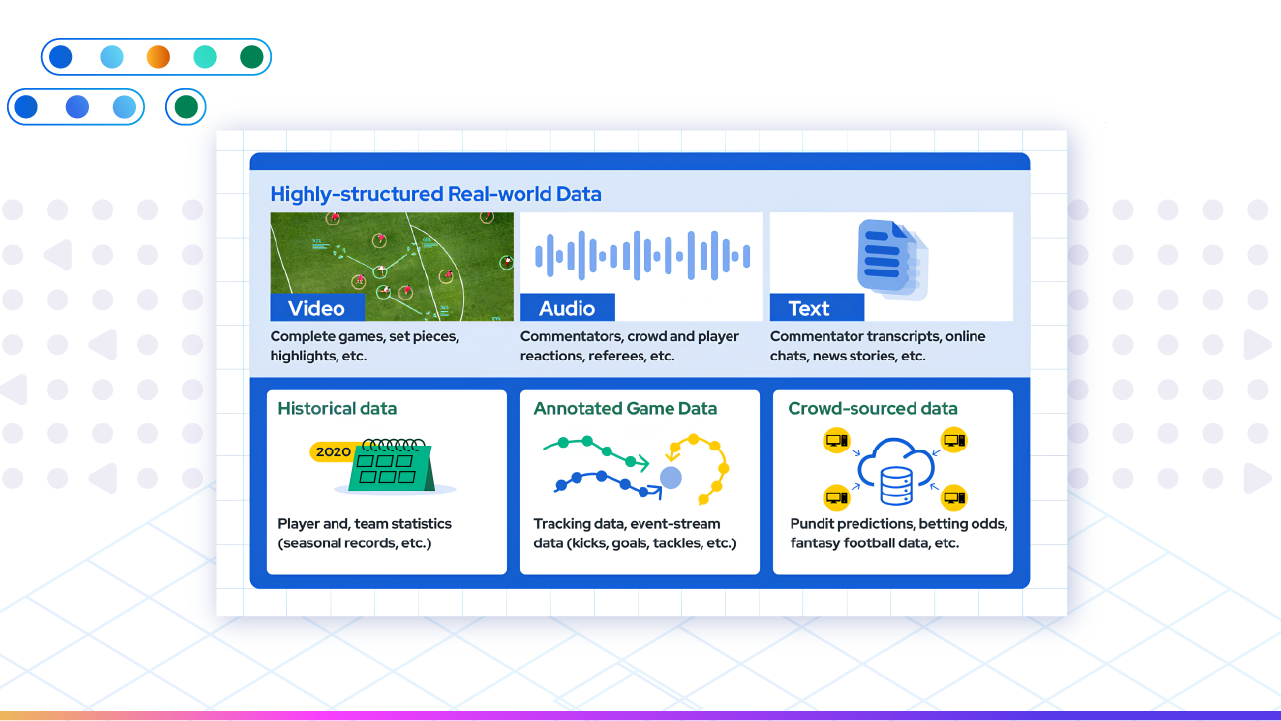
Injury prediction is a critical area within football analytics, aiming to forecast the likelihood of player injuries based on past and current season data. Previous research has explored the acute-chronic workload ratio (ACWR) as a predictor for sports-related muscular injuries. More recent studies have sought to leverage more prosperous workload measures obtained from Electronic Performance and Tracking Systems (EPTS) to predict future injuries using decision trees and manually designed features that comprehensively aggregate players’’ workload histories.
The human-centric nature of football analytics is emphasized by its focus on enhancing individual and team performance through predictive and prescriptive modelling, injury and fatigue prediction, and psychological analysis of players. This approach considers inter-player relationships, psychological challenges, and player discipline. It entails a comprehensive integration of research areas to evaluate the Impact of analytics on human play, which is inherently more complex than simulating and evaluating robotic agents.
AI in Football Analytics (Automated Video Assistant Coach)
Introduction: Football analytics has seen significant advancements, particularly with the introduction of multi-modal models utilizing various data types. However, manual data labeling and preparation remain time-consuming and costly. Our solution, the Automated Video Assistant Coach (AVAC), harnesses artificial intelligence (AI) to automate data collection, enabling coaches and decision-makers to focus on strategic development.
Key Features
1. Real-time Performance Analysis: AVAC observes players’ performance during games, identifying areas for improvement and offering personalized tips to enhance skills.
2. Pre-game Strategy Suggestions: Before matches, AVAC provides players and coaches with strategic insights tailored to exploit opponents’ weaknesses and defend against their strengths.
3. Player Position Evaluation: By evaluating performance in different playing positions, AVAC determines players’ optimal positions, aiding in team lineup decisions and player development.
Benefits for Coaches
1. Time Management: Coaches can efficiently manage their time with AVAC’s automated recommendations for team lineups, player scouting/trading, and game plan development.
2. Player Development: AVAC assists in identifying areas for improvement, facilitating targeted training sessions to enhance players’ skills and performance.
3. Strategic Advantage: With insights into opponents’ weaknesses and strengths, coaches can develop game plans to maximize their team’s chances of success.
Impact
1. Accessible Data Collection: AVAC automates data labeling and annotation, making football analytics more accessible and enabling the development of new learning algorithms.
2. Transformative Football Analytics: By reducing the reliance on manual data labeling, AVAC has the potential to revolutionize football analytics and support systems, driving innovation in the sport.
Conclusion
In conclusion, the integration of artificial intelligence (AI) technologies such as statistical learning, game theory, and computer vision has revolutionized football analytics, offering teams unprecedented insights into player performance, game strategies, and injury prevention. By leveraging predictive modeling, game theory analysis, and automated video processing, teams can make data-driven decisions to optimize their performance on the field.