Introduction
Contact centers serve as centralised hubs for consumer queries, feedback, providing support, assistance and other interactions across various channels. These centers typically rely on human agents who handle customer queries manually, addressing all kinds of interactions, resolving issues, and aiding based on predefined scripts and guidelines. Contact centers are crucial for organizations to maintain direct communication with customers and ensure high-quality service delivery.
Banks, owing to their huge customer base and the sheer volume of customer queries, need dedicated contact centres staffed with trained customer officers to handle inquiries and address concerns efficiently. These centres serve as points for customers seeking assistance with various banking services and transactions.
Contact Center Intelligence is the integration of AI and ML technologies within contact centres to make consumer interactions better, automate routine queries, predict issues, ensure security and compliance, and analyse real-time feedback for continuous improvement. They utilize technology like AI and analytics to improve efficiency and provide personalized customer experiences.Through continuous feedback analysis and proactive issue resolution, contact centres play a pivotal role in maintaining customer satisfaction and loyalty.
Personalized Customer Interactions
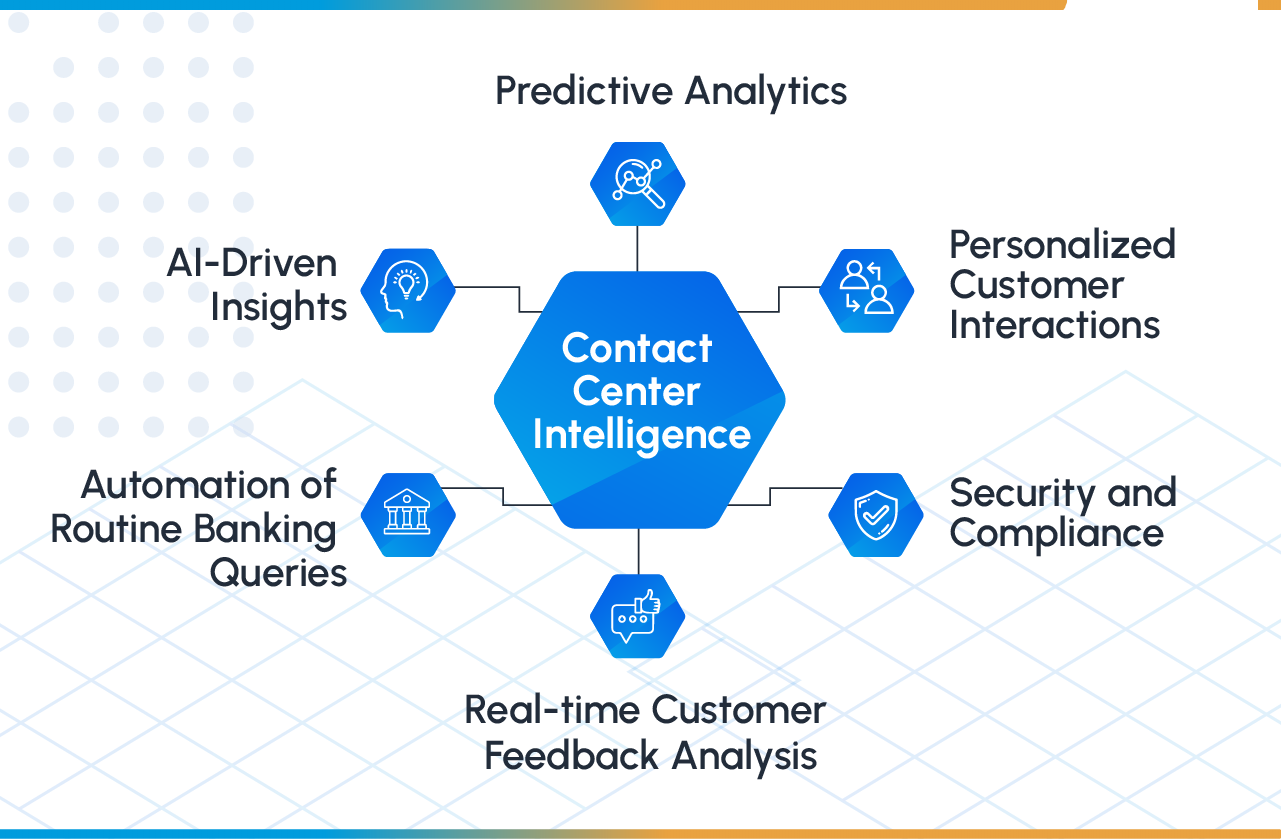
Integrating AI-driven insights enables contact centres to provide personalized consumer interactions by analysing huge datasets to understand individual preferences, behaviours, and needs. Through the utilization of AI technologies like natural language processing, generative AI, and machine learning, contact center agents can tap into real-time insights and historical data. This enables them to customize interactions, anticipate customer requirements, and provide pertinent solutions, ultimately improving the overall customer experience.
- Tailored Recommendations: AI-driven insights enable contact centres to analyse customer data and behaviours, allowing them to offer personalized product recommendations and services based on individual preferences.
- Predictive Analytics: Utilizing AI algorithms, contact centers can predict customer requirements and preemptively handle potential issues, resulting in smoother and more productive customer interactions.
- Contextual Understanding: AI technologies, such as natural language processing, enable contact centres to understand the context of customer inquiries, allowing agents to provide more relevant and helpful responses.
- Dynamic Scripting: AI-driven insights empower contact centre agents with dynamic scripting capabilities, enabling them to adapt their communication style and messaging based on real-time customer interactions and feedback.
- Customer Segmentation: AI-driven insights allow contact centres to segment customers based on various criteria, such as demographics, purchase history, and interaction preferences, enabling targeted and personalized communication strategies.
By leveraging AI, banks can analyse customer data to gain insights into preferences, behaviour, and needs. This enables personalized interactions, where agents can provide tailored solutions and recommendations based on individual customer profiles, enhancing overall satisfaction and loyalty.
Automating Routine Banking Queries
In today’s fast-paced banking landscape, the need for efficient and responsive customer service has never been greater. Automating routine banking queries with contact centre intelligence presents a transformative solution that streamlines operations, enhances customer experience, and drives operational efficiency. Through leveraging cutting-edge technologies like artificial intelligence and machine learning, banks can empower their contact centers to effectively manage routine inquiries, thereby liberating valuable human resources to address more intricate customer needs
- Self-Service OptionsContact centre intelligence automates routine banking queries by providing self-service options such as interactive voice response (IVR) systems and chatbots, allowing customers to access account information, make transactions, and resolve common issues without human intervention.
- Streamlined Processes: By leveraging AI-powered automation, contact centres can streamline the handling of routine queries, reducing wait times and improving overall efficiency. This allows agents to concentrate on intricate customer issues that necessitate human involvement, thereby improving the overall customer experience.
- 24/7 Availability: Contact centre intelligence enables banks to offer round-the-clock support for routine queries, ensuring that customers can access assistance whenever they need it, regardless of time or location. This improves accessibility and convenience for customers, leading to higher satisfaction levels.
- Quick Resolution: With automated processes in place, routine banking queries can be resolved quickly and accurately, minimizing customer frustration and reducing the need for follow-up interactions. This enhances operational efficiency and helps banks meet customer expectations for timely service.
- Data-driven Insights: Contact center intelligence gathers and analyzes data from customer interactions, providing banks with valuable insights into customer preferences, behavior patterns, and frequently asked questions. This information can be used to further optimize automated processes and improve the overall customer experience.
AI-driven automated chatbots and virtual assistants can manage routine banking inquiries like checking balances, viewing transaction histories, and updating accounts. This automation optimizes customer service processes, cuts down on wait times, and allows human agents to tackle more intricate issues that demand human intervention.
Predictive Analytics for Proactive Issue Resolution
Predictive analytics for proactive issue resolution is a game-changer in the banking industry, allowing banks to anticipate and address customer concerns before they escalate. By leveraging historical data, customer behaviour patterns, and machine learning algorithms, banks can predict potential issues and take pre-emptive measures to resolve them. Implementing this proactive strategy not only boosts customer satisfaction but also mitigates the impact of potential issues on business operations.
Predictive analytics enables banks to identify emerging trends, forecast customer needs, and implement proactive solutions, ultimately driving greater efficiency and improving the overall customer experience.
- Anticipating Account Fraud: Predictive analytics can identify suspicious patterns in account activity, such as unusual spending behaviour or login attempts from unfamiliar locations. As an illustration, banks employ machine learning algorithms to analyze transaction data and identify fraudulent activities in real-time, thwarting unauthorized access to accounts.
- Forecasting Customer Service Demand: By analysing historical call volumes, website traffic, and customer interaction data, banks can predict periods of high demand for customer service assistance. For instance, during peak times like holidays or product launches, predictive analytics can help banks allocate resources efficiently, ensuring timely support for customers.
- Preventing Payment Processing Issues: Predictive analytics can forecast potential issues with payment processing systems, such as transaction failures or delays. For instance, banks use predictive models to analyse transaction data and identify bottlenecks in the payment processing pipeline, allowing them to proactively address technical issues and minimize disruptions for customers.
- Early Detection of Loan Defaults: Predictive analytics can analyse borrower data and economic indicators to forecast the likelihood of loan defaults. For example, banks use machine learning models to assess the credit risk of loan applicants and identify customers who may struggle to repay their loans. By intervening early and aiding at-risk borrowers, banks can reduce the impact of loan defaults on their financial health.
- Proactive Maintenance of Banking Infrastructure: Predictive analytics can predict equipment failures or maintenance needs in banking infrastructure, such as ATMs or online banking platforms. For example, banks use sensor data and predictive models to anticipate equipment failures and schedule maintenance activities proactively, minimizing downtime and ensuring uninterrupted service for customers.
Using predictive analytics, banks can anticipate potential issues or trends based on historical data and customer behaviour patterns. This proactive approach enables contact centre agents to address concerns before they escalate, improving customer satisfaction and reducing the likelihood of negative experiences.
Security and Compliance Measures
AI-driven contact centers implement robust security measures to safeguard sensitive customer data and comply with industry regulations like GDPR (General Data Protection Regulation) and PCI DSS. These measures encompass encryption protocols, multi-factor authentication, and continuous monitoring for suspicious activities, ensuring the protection of customer information.
Security and compliance are paramount considerations in AI-powered contact centres within the banking sector. Here’s how banks ensure robust security and compliance measures:
- Data Encryption: To prevent unauthorized access or data breaches, all customer data, including personal and financial information, undergoes encryption both during transit and while at rest. Advanced encryption algorithms and secure communication protocols are utilized to safeguard sensitive data.
- Access Control: Role-based access control mechanisms are implemented to restrict access to customer data based on user roles and permissions. Only authorized personnel with appropriate credentials can access sensitive information, minimizing the risk of data misuse or unauthorized access.
- Regulatory Compliance: Banks follow stringent regulatory standards, including GDPR, CCPA, and PCI DSS, to maintain compliance with data protection and privacy regulations. Compliance frameworks are integrated into AI-powered contact centre solutions to facilitate regulatory audits and ensure adherence to legal requirements.
- Monitoring and Auditing: AI-powered contact centres incorporate robust monitoring and auditing mechanisms to track user activities, system interactions, and data access. Audit logs are maintained to provide a detailed record of user actions, facilitating forensic analysis and investigation in case of security incidents or compliance violations.
- Threat Detection and Prevention: AI-driven security solutions are employed to detect and mitigate potential security threats, such as malware, phishing attacks, or insider threats. Machine learning algorithms analyse network traffic patterns and user behaviour to identify suspicious activities and trigger proactive security measures.
- Continuous Security Updates: Contact centre systems are regularly updated with the latest security patches and software updates to address known vulnerabilities and mitigate emerging threats. Automated patch management processes ensure that security vulnerabilities are promptly addressed to maintain the integrity and security of the contact centre environment.
Overall, banks need to prioritize security and compliance in AI-powered contact centres to protect customer data, uphold regulatory requirements, and maintain trust and confidence in their services.
Real-time Customer Feedback Analysis
Real-time customer feedback analysis plays a pivotal role in driving continuous improvement initiatives within AI-powered contact centres in the banking sector. AI tools can analyse real-time customer feedback from various channels, including calls, emails, and social media, to identify trends, sentiment, and areas for improvement. This feedback loop enables banks to make data-driven decisions, refine service offerings, and enhance the overall customer experience over time. Here’s how banks leverage real-time feedback analysis for continuous improvement:
- Immediate Insights: Advanced analytics tools process customer feedback in real-time, extracting actionable insights from various sources such as surveys, social media, and customer interactions. This allows banks to promptly identify emerging trends, issues, or opportunities for improvement.
- Customer Sentiment Analysis: Natural language processing (NLP) algorithms analyse customer feedback to gauge sentiment and emotional responses. By understanding customer sentiments, banks can identify areas of dissatisfaction or delight and tailor their services accordingly to enhance customer experience.
- Performance Monitoring: Real-time feedback analysis enables banks to monitor the performance of contact centre agents, systems, and processes. Banks can pinpoint areas for optimization and efficiency improvement by monitoring key performance indicators (KPIs) like response time, resolution rates, and customer satisfaction scores.
- Proactive Issue Resolution: AI algorithms detect patterns in customer feedback, enabling banks to proactively address emerging issues or concerns before they escalate. By identifying recurring problems or pain points, banks can implement targeted solutions to improve service quality and prevent customer churn.
- Personalized Service Delivery: Real-time feedback analysis allows banks to gain insights into individual customer preferences, behaviours, and needs. By leveraging these insights, banks can personalize their interactions with customers, anticipate their requirements, and deliver tailored solutions to enhance satisfaction and loyalty.
- Continuous Learning and Adaptation: Utilizing AI-driven feedback analysis fosters continuous learning and adaptation in contact center environments.Banks can use feedback insights to refine their strategies, update processes, and train agents to better meet evolving customer expectations and market dynamics.
Overall, real-time customer feedback analysis serves as a valuable tool for banks to drive continuous improvement initiatives, enhance customer experience, and maintain competitiveness in the dynamic banking landscape.
Conclusion
Contact centre intelligence represents a transformative approach to customer service in the banking sector, leveraging advanced technologies to enhance efficiency, responsiveness, and customer satisfaction. With the advent of AI-powered solutions and platforms like AWS Connect, banks can unlock new capabilities for automating routine queries, predicting customer needs, and delivering personalized interactions. By harnessing real-time feedback analysis, banks can continuously refine their service offerings, address emerging issues proactively, and foster a culture of continuous improvement. As banks embark on the journey of implementing contact centre intelligence, key steps include assessing organizational needs and readiness, selecting appropriate technology solutions, integrating AI-driven analytics tools, training staff for AI adoption, and monitoring performance metrics for ongoing optimization. Through strategic investments in contact centre intelligence, banks can position themselves for long-term success in meeting the evolving expectations of customers and maintaining a competitive edge in the digital era.