Introduction of Generative AI in Data Security and Privacy Risks
The rise of generative AI presents many opportunities and challenges for businesses and organizations worldwide. While this technology can potentially revolutionize various sectors, its implementation poses multiple risks that must be addressed.
As we look ahead to the next few years, it is essential to develop comprehensive regulations and guidelines that account for the rapid pace of technological advancement and the broad capabilities of generative AI. By doing so, we can ensure that this technology is used safely, securely, and ethically while maximizing its benefits.
It is vital to continue monitoring the development of generative AI and explore its potential applications in various fields, including medicine, finance, and entertainment. Ultimately, the successful and efficient utilization of this technology will necessitate cooperation among various parties involved, such as policymakers, industry professionals, and consumers.
Threat Actors in the Age of Generative AI
- The proliferation of Threat Vectors: The growing performance, availability, and accessibility of generative AI tools raises concerns as virtually anyone could pose a threat through malicious intent, misuse, or unintended incidents. The expanding capabilities of generative AI are likely to reduce entry barriers, enabling less sophisticated threat actors, including organized groups, political activists, and lone actors, to leverage generative AI for ideological, political, or personal purposes.
- Criminal Adoption and Innovation: Criminals are anticipated to adopt generative AI technology at a pace mirroring the general population, with certain innovative groups and individuals being early adopters. This adoption is expected to contribute to an increase in the frequency and sophistication of various illicit activities, including scams, fraud, impersonation, ransomware, currency theft, data harvesting, child sexual abuse imagery, and voice cloning. However, until 2025, criminals may encounter challenges in exploiting generative AI to create novel malware.
- Terrorist Utilization of Generative AI: By 2025, generative AI can potentially enhance terrorist capabilities across multiple domains, including propaganda, radicalization, recruitment, funding streams, weapons development, and attack planning. Nonetheless, reliance on physical supply chains will likely hinder the extensive use of generative AI for sophisticated physical attacks.
Safety and security risks
Over the next 18 months (about one and a half years), generative AI is more likely to amplify existing risks than create new ones. But it will increase sharply the speed and scale of some threats and introduce some vulnerabilities. The risks fall into at least three overlapping domains:
- Digital risks are assessed to be the most likely and have the highest impact in 2025. Threats include cybercrime and hacking. Generative AI will also improve digital defenses against these threats.
- Risks to political systems and societies will likely increase in 2025, becoming as significant as digital risks as generative AI develops and adoption widens. Threats include manipulation and deception of populations.
- Physical risks will likely rise as generative AI becomes embedded into more physical systems, including critical infrastructure and the built environment. If implemented without adequate safety and security controls, AI may introduce new risks of failure and
Emerging Risks of Generative AI by 2025
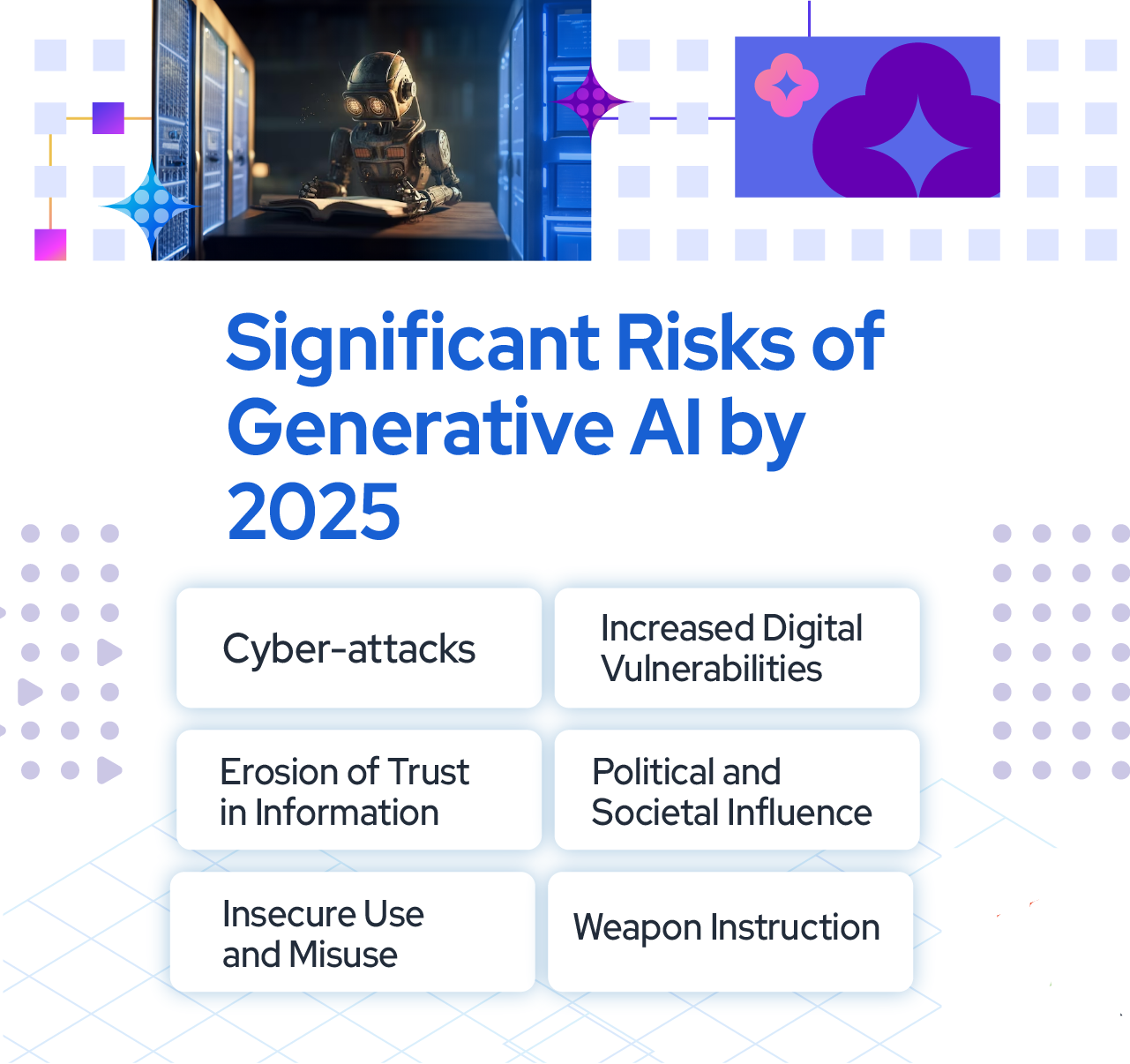
- Cyber-attacks: Generative AI poses the risk of enabling faster, more effective, and larger-scale cyber intrusions through tailored phishing methods and replicating malware. While vulnerability discovery and evasion experiments are less mature, complete automation of computer hacking via generative AI is deemed unlikely by 2025.
- Increased Digital Vulnerabilities: Integration of generative AI into critical functions and infrastructure introduces new attack vectors, such as corrupting training data (data poisoning), hijacking model output (prompt injection), extracting sensitive training data (model inversion), misclassifying information (perturbation), and targeting computing power.
- Erosion of Trust in Information: Generative AI could contaminate the public information ecosystem with hyper-realistic bots and synthetic media (deepfakes), influencing societal debate and reflecting existing social biases. This risk encompasses the creation of fake news, personalized disinformation, market manipulation, and undermining the criminal justice system. By 2026, synthetic media may constitute a significant portion of online content, potentially eroding public trust in government and increasing polarization and extremism. Authentication solutions like ‘watermarking’ are in development but must be more reliable.
- Political and Societal Influence: Generative AI tools have showcased their ability to influence individuals on political matters, magnifying the extent, persuasiveness, and frequency of false information and misleading content. Moreover, generative AI can generate hyper-targeted content with unprecedented scale and sophistication.
- Insecure Use and Misuse: Incorporating generative AI into essential systems and infrastructure heightens the potential for data breaches, partial and prejudiced systems, or compromised human decision-making. Inappropriate use by large-scale organizations could have unintended consequences, leading to cascading failures. Over-reliance on opaque and potentially fragile supply chains controlled by a small number of firms is also a concern.
- Weapon Instruction: Generative AI can be exploited to assemble knowledge on physical attacks by non-state violent actors, including creating chemical, biological, and radiological weapons. While leading generative AI firms are implementing safeguards, barriers to entry, such as acquiring components and manufacturing equipment, persist, albeit potentially accelerated by generative AI trends.
Best Practices for Maintaining Data Privacy within AI
- Blockchain-Enabled Data Lineage: Utilize blockchain technology to establish an unmodifiable chain that ensures the traceability and origin of data. Blockchain’s cryptographic storage and sequential protection of data alterations facilitate robust auditing capabilities. This technology provides a foundation for advanced data governance tools, showcasing their inflexibility to regulatory authorities.
- Lifecycle Management: It is imperative for organizations to possess expertise in lifecycle management in order to provide AI-enabled systems with essential data and eliminate outdated data. Implement expiration periods for data in adherence to data governance policies, ensuring compliance with legal data protection requirements. Organizations should take responsibility for the timely destruction of expired data stores, demonstrating a commitment to retention periods.
- Security and Disaster Recovery: Employ advanced technologies akin to blockchain for securing data against viruses and malware programs. Classify stored data into different tiers, allowing for replacing data tiers with shortcuts after a specified time, creating space for new data. Automate the creation of shortcuts to enable quick and intelligent data restoration in the event of security threats, such as ransomware attacks. Data archiving systems with disaster recovery capabilities can efficiently restore systems, providing a systematic recovery process after catastrophic events. This approach ensures the seamless restoration of critical business functions.
Conclusions of Data Security and Privacy Risks of Generative AI
Generative AI has the potential to uplift productivity and innovation in various sectors, but inadequate understanding of the technology could lead to disproportionate public anxiety and hinder its adoption. Generative AI will also increase safety and security risks by enabling threat actors to enhance their capabilities and launch more sophisticated attacks. Governments may need more insight into private sector progress, making mitigating all safety and security risks challenging. Moreover, the race to develop the best-performing generative AI models will intensify, and it could accelerate the development of other technologies such as quantum computing, novel materials, telecommunications, and biotechnologies. However, this convergence will likely increase risks beyond 2025.