- Advanced search capabilities enhance knowledge management by offering context-aware, dynamic, and intuitive search results.
- AI-driven systems continuously learn from user interactions, improving search accuracy and relevance over time.
- Traditional KM methods struggle with inefficiency, siloed data, and rigid structures, highlighting the need for smarter, adaptive search solutions.
Imagine a global organization drowning in its own data. Despite housing vast reservoirs of critical information, employees struggled to locate the right insights when needed most. Time-intensive searches, irrelevant results, and fragmented knowledge silos hampered collaboration, decision-making, and innovation.
This was the reality for many companies relying on traditional Knowledge Management (KM) systems. Manual categorization and keyword-based searches, once sufficient, now fall short in handling the growing complexity of modern data. Agentic AI-driven Knowledge Management with Advanced Search Capabilities (ASC) transforms how businesses manage information.
We find out how AI Agents transform knowledge into actionable insights, empowering organizations to innovate and thrive.
What is Knowledge Management for Advanced Search Capabilities?
Knowledge Management (KM) is the systematic process of creating, sharing, using, and managing organizational knowledge and information. In the context of Advanced Search Capabilities (ASC), KM encompasses advanced methodologies and tools that organize, retrieve, and utilize information from diverse data sources while maintaining context and relevance.
The integration of KM with ASC establishes a framework where information is not just stored but is discoverable through intelligent search mechanisms that understand context, intent, and interrelationships between various pieces of information. This synergy enables organizations to harness their data assets efficiently, leading to better decision-making and innovation.
Key Concepts of Knowledge Management for Search Capabilities
- Enhanced Context Understanding: Focuses on interpreting user intent and query meaning rather than relying solely on exact keywords, delivering highly relevant results.
- Dynamic Information Organization: Adapts data categorization to align with evolving organizational structures, ensuring information remains accessible and up-to-date.
- Interconnected Knowledge: Uses knowledge graphs to reveal data relationships, providing a comprehensive view and uncovering deeper insights.
- User-Centric Search: Employs AI and NLP to create personalized, context-aware search experiences, making information retrieval intuitive and efficient.
- Scalable Solutions: Designs systems to handle expanding data volumes seamlessly, maintaining speed, accuracy, and performance at scale.
Traditional Approaches to Knowledge Management for Advanced Search Capabilities
Historically, Knowledge Management (KM) practices were based on manual processes and rigid systems to organize and retrieve organizational knowledge. While functional in the past, these methods struggle to meet the complex needs of modern users. Here’s an overview of key traditional approaches:
- Manual Categorization: Human effort sorts information into predefined folders and categories. This labour-intensive approach is prone to errors, and as data grows, managing categories becomes inefficient, leading to inconsistencies in information retrieval.
- Keyword-Based Search: Traditional search systems rely on exact keyword matches within documents or metadata. However, they don’t account for user intent or context, often returning irrelevant or incomplete results, which can frustrate users.
- Rigid Taxonomies: Traditional KM systems use fixed taxonomies to categorize information. While structured, these taxonomies are inflexible and require manual updates to adapt to organizational needs, slowing the system’s evolving ability.
Impact on Customers Due to Traditional Approaches
The limitations of conventional KM methods significantly affect end-users, resulting in a less efficient and often frustrating experience. Here’s how these challenges manifest:
- Time Consumption: Users waste time searching through disorganized or poorly indexed data. Without advanced search capabilities, refining searches and sifting through irrelevant results becomes cumbersome, lowering productivity and morale.
- Information Silos: Knowledge often remains trapped within specific departments or systems, limiting cross-team access to crucial information. This fragmentation hinders collaboration, slows decision-making, and leads to duplicated efforts across the organization.
- Inconsistent Results: Search outcomes depend on exact keyword matches, requiring users to guess the precise terminology. Minor variations in phrasing or spelling can result in incomplete or irrelevant results, causing frustration and diminishing trust in the system.
- Limited Context: Traditional systems can’t understand user intent or the context of queries. This leads to irrelevant search results and forces users to conduct multiple searches or manually piece together insights, slowing decision-making.
- Outdated Information: Manual updates to knowledge bases are slow, causing information to become obsolete or inaccurate. Users may unknowingly rely on stale data, resulting in errors, poor decisions, and reduced confidence in the system’s reliability.
These challenges emphasize the need for more advanced solutions that can deliver precise, context-aware, and timely information to users.
Agentic AI: Multi-Agent in Action
AI agents simplify and enhance Knowledge Management by automating tasks that ensure efficiency, accuracy, and scalability.
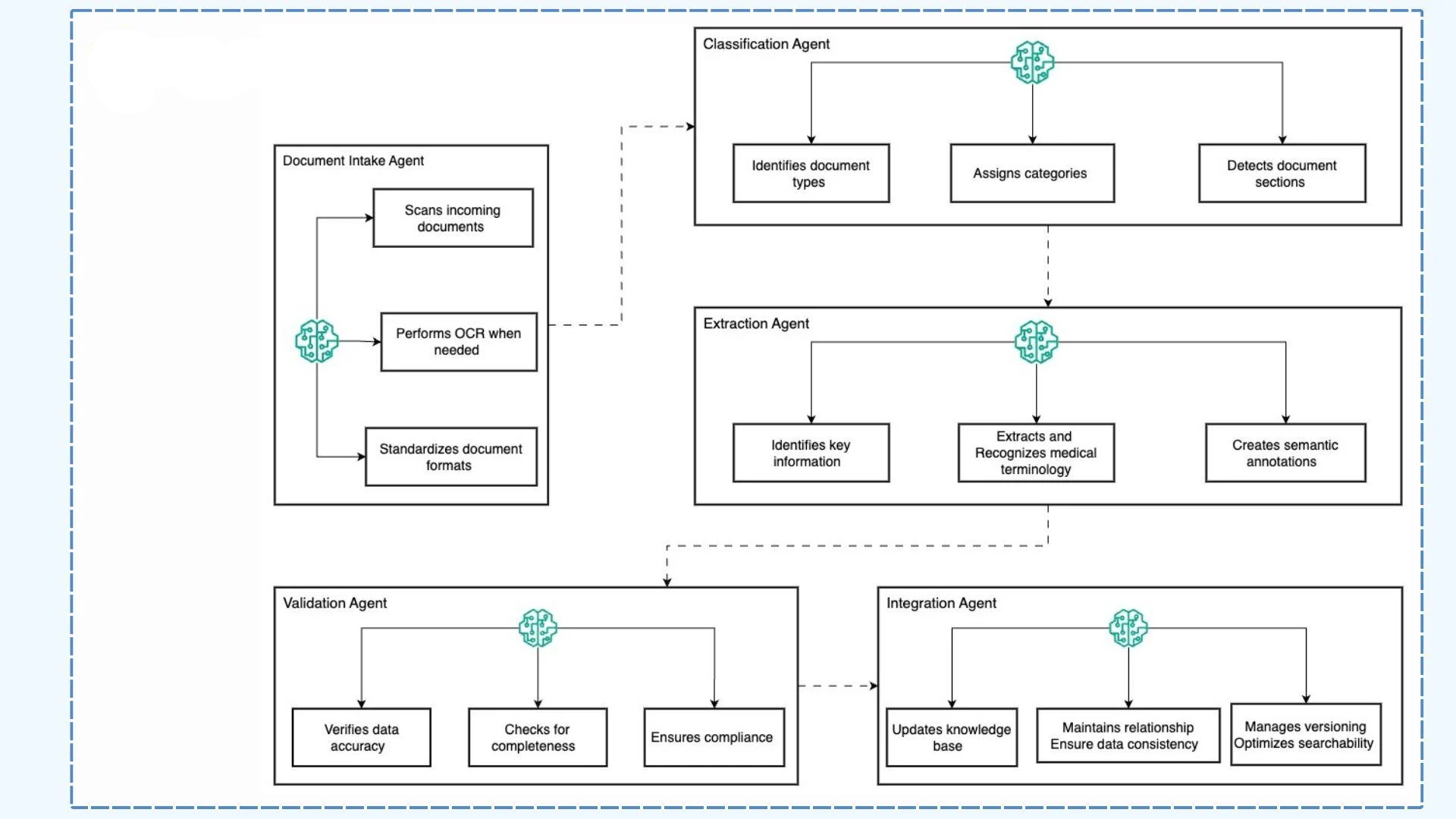
- Document Digitization and Standardization: AI agents convert physical documents into digital formats using Optical Character Recognition (OCR) technology, capturing text and data with precision. They also standardize formats (e.g., PDFs, Word files) to ensure compatibility across systems, making the documents universally accessible and ready for advanced processing.
- Automated Classification: Classification agents analyze the content of documents to categorize them accurately. Whether it’s identifying the document type (e.g., invoices, contracts, reports) or assigning tags based on topics or keywords, this step ensures that the data is organized systematically. This minimizes human error and improves retrieval efficiency.
- Information Extraction: Specialized AI agents extract crucial details such as names, dates, locations, and relationships between data points. They create semantic annotations, ensuring that information isn’t just stored but is also enriched with context. This deeper understanding helps uncover insights that would otherwise remain hidden in raw data.
- Validation and Quality Assurance: Validation agents play a critical role in ensuring data reliability. They cross-check extracted information for accuracy, verify compliance with organizational and regulatory standards, and flag inconsistencies or errors. This step builds trust in the system and ensures high-quality outputs.
- Integration and Optimization: It integrates processed information into knowledge bases, connecting data points and maintaining consistency. They also optimize searchability by updating relationships, ensuring users can easily find and utilize relevant information. This step aligns the entire knowledge ecosystem with the organization’s evolving needs.
Together, these steps create a seamless and intelligent workflow, transforming raw data into a strategic asset that drives productivity and informed decision-making.
Prominent Technologies in Advanced Search Capabilities
Several advanced technologies have emerged to address Knowledge Management (KM) challenges and enhance Advanced Search Capabilities (ASC), enabling more efficient information management and improved search accuracy. Here are some key technologies:
- Enterprise Search Platforms: Tools like Apache Solr and Elasticsearch index large volumes of content quickly, enabling fast, scalable searches across multiple data sources for enhanced KM efficiency.
- Document Management Systems (DMS): Platforms such as SharePoint and Alfresco centralize storage, enhance collaboration, and ensure version control, improving access to and sharing of documents.
- Text Analytics Tools: Tools like IBM Watson and OpenText analyze unstructured text, extract key insights, and identify trends, providing deeper understanding and better decision-making.
- Knowledge Graph Databases: Databases like Neo4j and Amazon Neptune map relationships between data, helping visualize complex connections and improving search relevance.
- Natural Language Processing (NLP) Systems: NLP models like BERT and GPT interpret queries with context and intent, improving search accuracy and user interaction with KM systems.
How AI Agents Supersede Technologies in KM
AI agents bring transformative capabilities to Knowledge Management (KM) for Advanced Search Capabilities (ASC), offering several key advantages over traditional technologies. These intelligent systems enhance efficiency, accuracy, and adaptability in ways that older methods cannot. Here’s how AI agents outperform traditional systems:
- Autonomous Learning: The system continuously improves based on user interactions, refining search results with each query. Over time, this leads to more accurate and relevant outcomes, unlike traditional systems that remain static.
- Dynamic Adaptation: The system adapts to new data and changing user needs in real time, ensuring access to the most relevant and up-to-date content. Unlike traditional systems, which can be rigid, this flexibility helps organizations stay current and responsive.
- Context Understanding: By interpreting user intent and query context, the system delivers more accurate, context-aware results. This allows for more intuitive and effective searches, surpassing the limitations of simple keyword-based searches.
- Intelligent Automation: Routine tasks like tagging, categorization, and metadata generation are automated, reducing manual effort and errors. This streamlines workflows and ensures a more consistent and organized knowledge base.
- Predictive Insights: The system analyzes past behaviours to predict user needs, proactively suggesting relevant content. This reduces search time and enhances decision-making by providing valuable information before it’s actively needed.
Successful Implementations of AI Agents in Search Capabilities
AI agents have significantly transformed Knowledge Management (KM) systems in various industries, particularly healthcare. Below are some successful examples:
- Siemens: Siemens utilized machine learning and natural language processing to enhance data retrieval across its engineering departments. This innovation helped employees find complex technical documents faster, reducing searching time and boosting productivity.
- Shell: Shell adopted semantic search to better manage its vast library of technical papers and manuals. By understanding the meaning behind user queries, the system enabled faster and more accurate access to crucial information, streamlining workflows and improving decision-making.
- Unilever: Unilever improved supply chain management using an intelligent search system that quickly pulled up inventory data and supplier records. This optimized procurement processes and minimized delays, resulting in better operational efficiency.
- General Electric (GE): GE enhanced its industrial operations by providing real-time access to manuals, designs, and maintenance records. The system’s contextual understanding helped engineers resolve issues faster, reducing downtime and increasing productivity.
- Walmart: Walmart implemented a smart search system to help employees quickly access product information, inventory levels, and sales data. This improved inventory management and boosted customer service by providing real-time insights to meet customer demands.
Transforming Business Operations Through Smarter Search
In conclusion, advanced search capabilities are reshaping how organizations manage and access knowledge. By moving beyond traditional methods, businesses can streamline information retrieval, improve decision-making, and enhance productivity. These systems provide more accurate, relevant, and context-aware results, making it easier for employees to find what they need quickly.
This leads to faster workflows, improved collaboration, and better customer experiences. As organizations continue to adopt these enhanced search solutions, they will be better equipped to manage growing data volumes, remain agile, and stay competitive in an increasingly fast-paced business environment.