- Agentic AI-driven predictive maintenance uses real-time data and machine learning to forecast equipment failures, reducing unplanned downtime and optimizing resources.
- The shift from traditional reactive strategies to proactive, data-driven maintenance boosts system reliability. It extends asset lifespan.
- AI agents streamline maintenance processes, driving cost savings, improving operational efficiency, and transforming infrastructure management across industries.
In industrial automation, a leading manufacturing plant faced recurring equipment failures and escalating maintenance costs due to traditional reactive strategies. Struggling to keep up with downtime and inefficiencies, the company turned to AI-driven predictive maintenance. By leveraging AI agents, they shifted from costly, reactive repairs to proactive, data-driven strategies, minimizing unplanned downtime, optimizing resources, and enhancing system performance.
This transformation in industrial automation highlights how AI Teammates are revolutionizing maintenance processes. This allows businesses to streamline operations, drive significant cost savings, and improve productivity.
What is Predictive Maintenance of Critical Infrastructure?
Predictive maintenance is a proactive strategy that leverages real-time data, advanced analytics, and machine learning to predict equipment failures before they occur. By continuously monitoring the condition of critical infrastructure through sensors and data collection, PdM analyzes patterns and trends to identify early signs of potential issues.
This approach helps optimize maintenance schedules, reduce downtime, and extend the lifespan of assets. By relying on data-driven insights and economical computing power, predictive maintenance ensures the reliable operation of essential systems. It is a key component in the manufacturing, energy, transportation, and telecommunications industries.
Key Concepts of Predictive Maintenance for Critical Infrastructure
- Real-Time Data Collection: Continuous monitoring of equipment using sensors captures crucial data, such as temperature, vibration, and pressure, allowing for the timely detection of potential issues.
- Data Analytics and Machine Learning: Advanced analytics and machine learning algorithms analyze the collected data, identifying patterns and anomalies that predict potential failures before they occur.
- Failure Prediction: Predictive models forecast equipment failures by analyzing historical and real-time data, enabling proactive maintenance and reducing unexpected downtime.
- Condition Monitoring: Regular assessment of equipment health through real-time data ensures that assets are operating efficiently, with maintenance triggered only when necessary.
- Cost Optimization: By reducing unplanned downtime and extending asset lifespan, predictive maintenance helps organizations save on repair costs, improve resource allocation, and maximize operational efficiency.
Traditional Way of Predictive Maintenance for Critical Infrastructure
The traditional predictive maintenance approach for critical infrastructure often relies on scheduled inspections, manual checks, and condition-based monitoring. Maintenance activities are typically performed at fixed intervals, regardless of the equipment’s actual condition, which can result in missed early warning signs of potential failures. Data collected from infrastructure is often analyzed manually or using essential software tools, with human experts interpreting the results based on experience rather than advanced predictive models.
Repairs or replacements are usually conducted reactively after a failure, leading to unexpected downtime and increased repair costs. Additionally, traditional methods lack scalability, requiring significant manual intervention, making them resource-intensive and less effective for large or complex infrastructure systems. While functional, this approach tends to be inefficient, costly, and prone to delays, especially when dealing with modern, interconnected infrastructure.
Impact on Customers Due to Traditional Predictive Maintenance Methods
- Unplanned Downtime: Traditional maintenance methods can cause unexpected equipment failures, leading to service interruptions. This results in delays or complete outages, disrupting normal operations.
- Increased Maintenance Costs: Reactive repairs after breakdowns often involve higher costs due to emergency fixes and expedited parts. These increased expenses can strain budgets and resources.
- Lower Equipment Reliability: Traditional methods often result in frequent breakdowns and unreliable equipment without continuous monitoring, which leads to more disruptions and reduced performance.
- Service Disruptions: Maintenance schedules may not align with actual equipment conditions, causing unexpected service interruptions. This can hinder the overall efficiency and reliability of infrastructure.
- Financial Losses and Safety Risks: Unplanned failures lead to financial losses from repair costs and downtime. In critical sectors, these failures also pose safety risks, further escalating costs and hazards.
Agentic AI: Multi-Agents in Action
AI agents can analyze data at multiple stages within the infrastructure system to ensure predictive, highly efficient, and reliable maintenance. These analysis levels enhance the effectiveness of maintenance strategies by targeting different aspects of system performance and health.
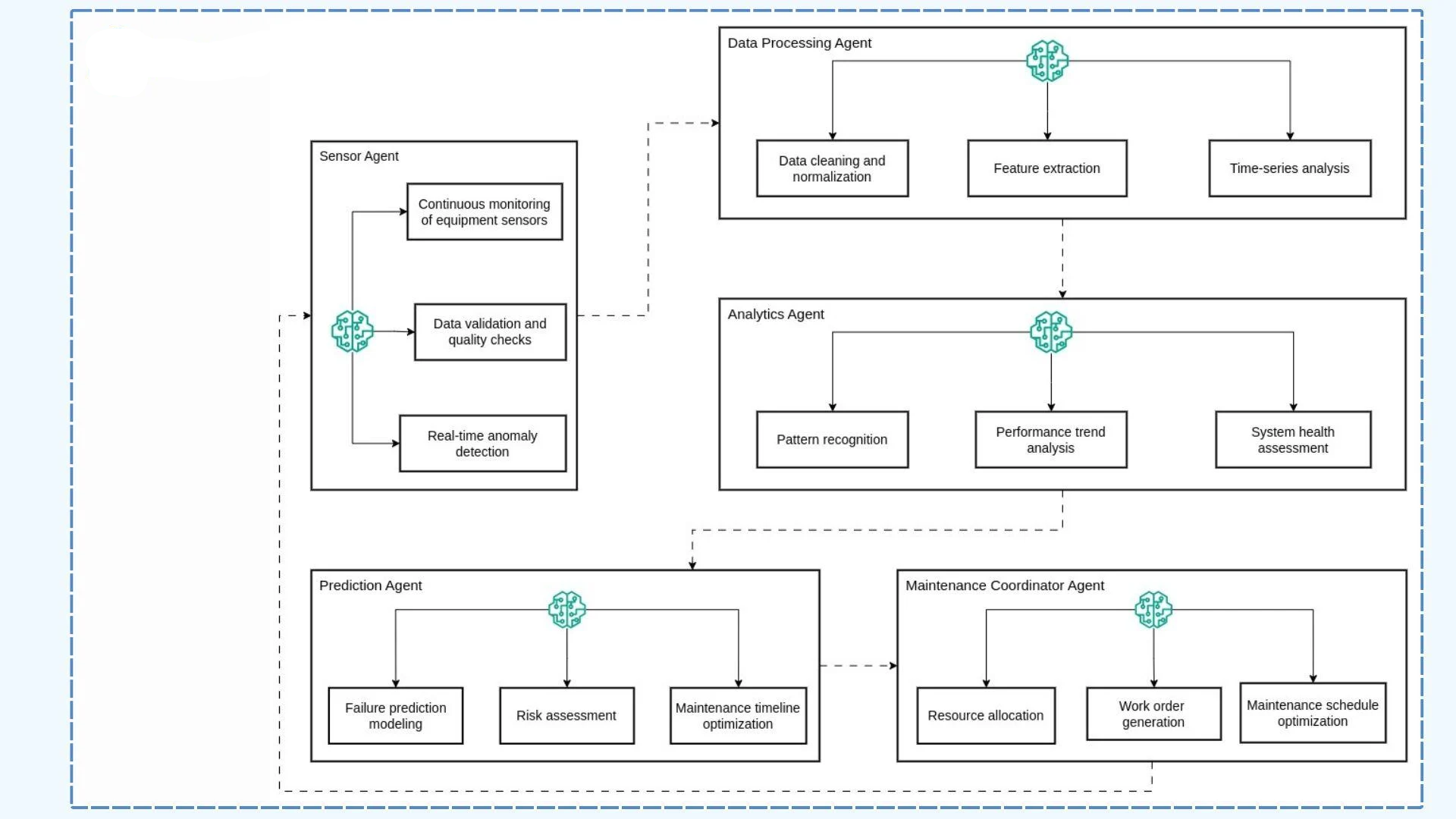
- Sensor Agent: The Sensor Agent connects physical infrastructure to the AI system, gathering real-time data from various sensors, such as temperature and vibration. It ensures the accuracy and reliability of the collected data, providing the foundation for effective monitoring and analysis.
- Data Processing Agent: The Data Processing Agent converts raw sensor data into structured information by cleaning and normalizing it. It handles missing data and ensures consistency, preparing the information for further analysis to drive accurate decision-making.
- Analytics Agent: The Analytics Agent analyzes the processed data using advanced algorithms to detect patterns, trends, and correlations in system performance. It identifies potential issues by recognizing anomalies and signs of deterioration, enabling early intervention before failures occur.
- Prediction Agent: The Prediction Agent uses machine learning models to forecast future failures and estimate the remaining useful life of the equipment. Leveraging historical and real-time data predicts when maintenance should be performed to avoid unexpected breakdowns.
- Maintenance Coordinator Agent: The Maintenance Coordinator Agent organizes and schedules maintenance activities based on the predictions provided by the Prediction Agent. It optimizes resource allocation, ensuring the right personnel and tools are available when needed, reducing unnecessary downtime and operational disruptions.
This multi-agent analysis ensures a holistic view of infrastructure health, enabling proactive decision-making and extending the operational lifespan of critical systems.
Prominent Technologies in the Space of Predictive Maintenance
Several advanced technologies contribute to the success of predictive maintenance strategies by enabling AI agents to provide accurate, real-time insights and proactive decision-making. These technologies include:
- Machine Learning Algorithms: Machine learning algorithms like neural networks and random forests analyze complex data patterns to predict failures and optimize maintenance schedules. These models improve over time, offering increasingly accurate predictions for equipment life and potential issues.
- IoT Sensors: IoT sensors collect real-time data such as temperature, pressure, and vibrations from equipment, providing detailed insights into system conditions. This data helps AI agents detect early signs of failure, enabling proactive maintenance actions.
- Cloud Computing: Cloud platforms store and process large volumes of data from IoT sensors, facilitating real-time data analysis and collaboration. They also support deploying machine learning models, ensuring scalable and efficient predictive maintenance across distributed systems.
- Digital Twin Technology: Digital twins create virtual replicas of physical assets, simulating real-world conditions to monitor, predict, and analyze system behaviour. They help identify potential failures and optimize maintenance strategies by testing scenarios in a virtual environment before physical implementation.
- Edge Computing: Edge computing processes data near its source, reducing latency and enabling real-time analysis. This allows for immediate detection of anomalies and quick responses to emerging issues without waiting for cloud processing, which is essential for critical infrastructure systems.
How AI Agents Supersede Other Technologies in Predictive Maintenance
AI agents significantly improve predictive maintenance over traditional technologies by combining advanced algorithms, real-time analysis, and autonomous decision-making. Here’s how they outperform other systems:
- Enhanced Predictive Capabilities: Advanced machine learning processes large datasets to identify emerging patterns and predict equipment failures more accurately than traditional methods. Early detection minimizes unplanned downtime, allowing for optimized maintenance schedules and cost savings.
- Faster Response Times: Continuous real-time monitoring ensures immediate detection of anomalies, triggering swift alerts. This quick response prevents minor issues from escalating, reducing the risk of significant disruptions and enabling timely corrective actions.
- Comprehensive Insights: Data from multiple sources is analyzed to provide a holistic view of infrastructure health. This broad analysis detects complex, system-wide issues that might be missed by traditional methods, supporting informed decision-making.
- Autonomous and Collaborative Tasks: Routine tasks such as monitoring, data collection, and analysis are performed autonomously, minimizing human intervention. Collaborative workflows ensure tasks are efficiently managed, with each system component handling its specific responsibilities.
- Continuous Learning and Adaptation: Predictive models improve over time by learning from fresh data and adapting to evolving conditions. This adaptive capability ensures highly accurate predictions, while traditional methods often require manual updates to stay relevant.
Successful Implementations of AI Agents in Critical Infrastructure
Several industries have successfully implemented AI agents for predictive maintenance, demonstrating the technology’s potential to revolutionize infrastructure management:
- Power Grids: Duke Energy utilizes AI-powered systems to monitor their power grid, mainly focusing on transmission lines and transformers. These systems predict potential failures, reducing the likelihood of outages. For instance, AI models analyze real-time data from IoT sensors embedded in grid components to foresee electrical disruptions, allowing the company to address issues proactively and ensure continuous power supply.
- Transportation Networks: Hitachi has integrated AI into its rail network maintenance system to predict potential failures in tracks, bridges, and signal equipment. Their predictive maintenance system uses AI Agents to monitor real-time data from sensors on trains and infrastructure, forecasting possible breakdowns.
- Water Supply Systems: Veolia, a global leader in optimized resource management, uses AI to monitor water distribution networks. AI systems detect leaks, pressure drops, and contamination risks in real-time by processing data from sensors placed in pipes. This predictive maintenance helps prevent water loss, improve service reliability, and ensure water quality for consumers.
- Manufacturing: Siemens uses Agentic AI-driven predictive maintenance in its manufacturing plants, where AI analyzes sensor data from machinery to predict failures. This system allows manufacturers to schedule repairs before machinery breaks down, optimizing equipment uptime and minimizing unplanned downtime. For example, AI systems can forecast when a motor will fail and schedule a replacement, avoiding costly disruptions to the production process.