In a cutthroat market for consumer products, getting them to the consumers on time, when and where they need them, and at the correct cost becomes critical. However, supply chains have become sophisticated today based on several attributes, such as demand volatility, geopolitics, and a global environment. Many traditional supply chain management approaches are not practical enough in these terms to respond to such challenges promptly and adequately.
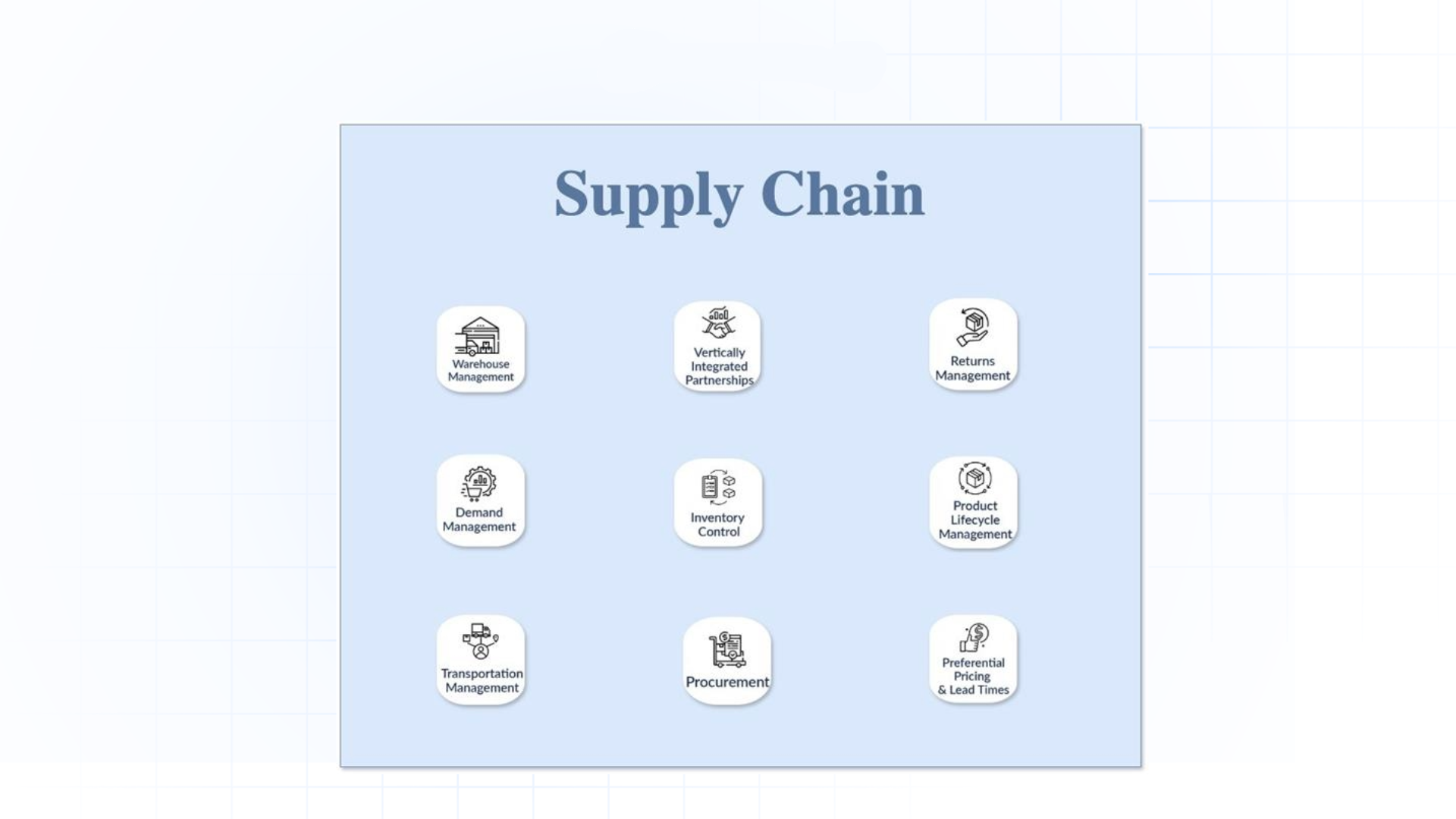
Introducing predictive analytics – an innovative solution based on data science, machine learning and AI. The use of Big Data methods helps organizations analyze historical and current data and apply the results to make a range of decisions based on the likely future outcomes.
The Consumer Goods industry opens new opportunities in demand forecasting, inventory management, transportation and risk management.
What does Predictive Analytics Mean in the Supply Chain?
PA is the application of statistical models and machine learning, big data that forecasts outcomes with high accuracy. Through analysis of patterns, relationships, and exceptions, it helps businesses forecast periods of increasing needs and supply, transportation, and other essential activities.
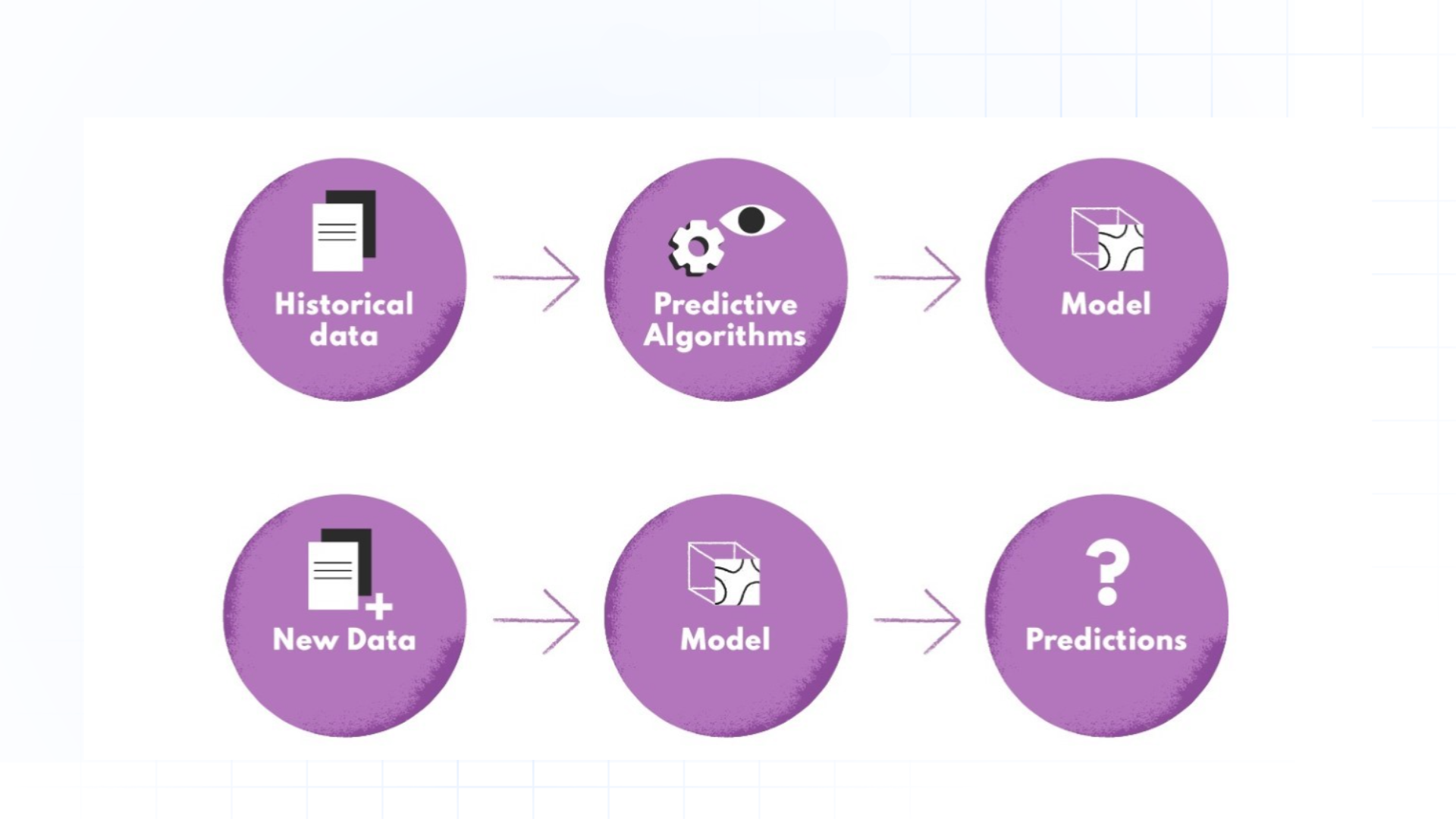
Why Predictive Analytics is an absolute must for Consumer Products Company
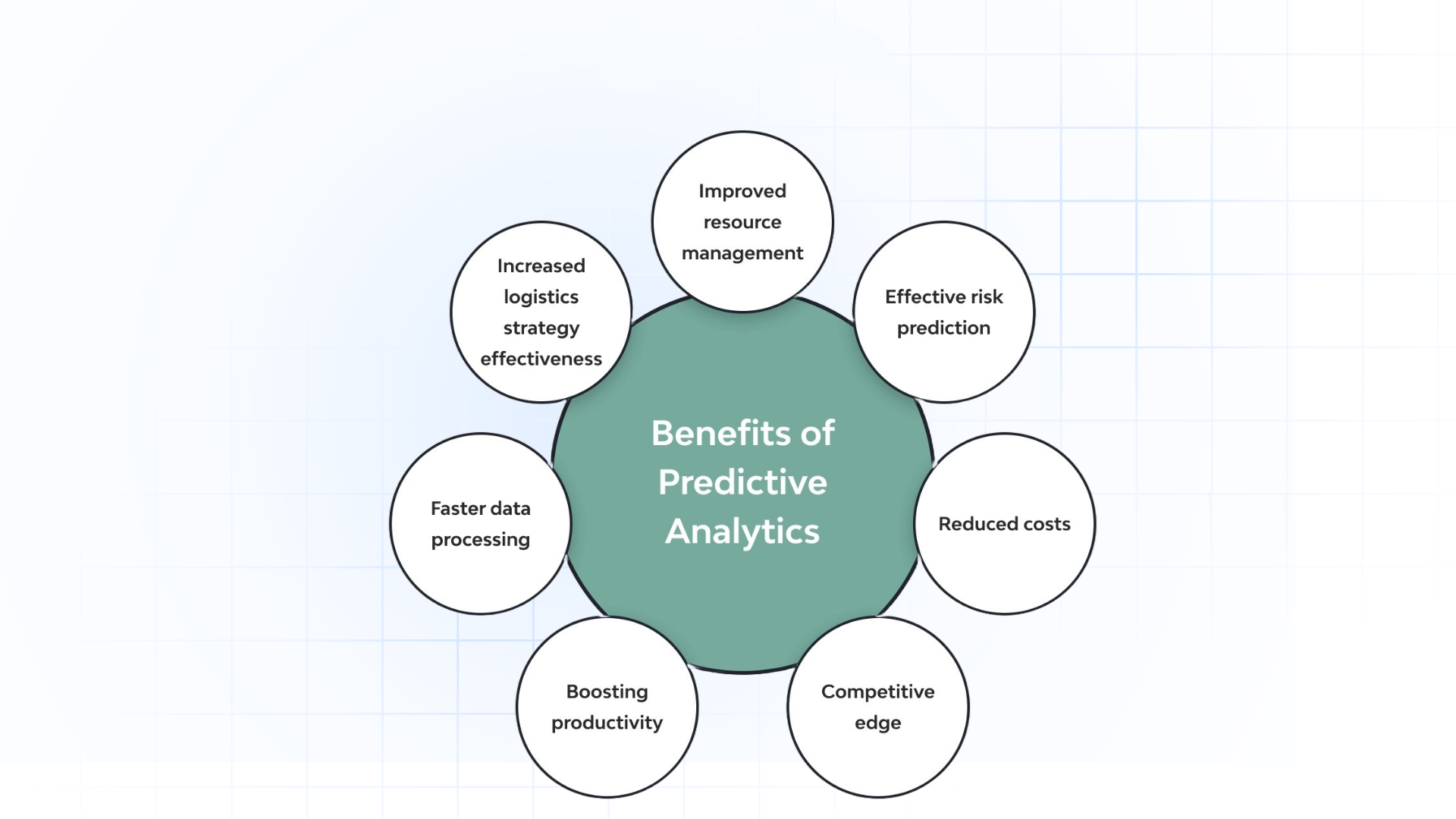
- Tackling Demand Volatility Consumer goods demand depends on the season, the economic conditions of the state, and changes in consumer behaviour trends. Forecasting enables business organizations to prevent stockouts or overstocking by showing tendencies in demand.
- Measures in Managing Supply Chain Disruptions Natural disasters threaten global supply chains, changes in the political environment, and supplier-related problems. Risk assessments can be predicted using predictive models to determine contingency measures to reduce disruptions to operations.
- Increasing organizational efficiency Predictive analytics for fixed assets also make it possible to define inefficiencies and sources of delays in the supply chain and workflow and then plan how best to optimize activities that cause delays in the supply chain, hence minimizing lead time and costs.
- Improving the Quality of Customer Experience Sales demand forecasting guarantees customer satisfaction because a product is used when required by a customer, thereby leading to consumer loyalty.
General Uses of Predictive Analytics for Supply Chain Management
1. Demand Forecasting: Selling forecasting redesign is one of the key benefits of predictive analytics, where historical sales data, market demand, and conditions that might influence the market, for instance, weather conditions or celebrations, are analyzed. On a detailed level, using AI tools, demand can be predicted at the segment level down to the regional, store or even product level.
Example: Large retailer Walmart incorporates predictive models to forecast fluctuations during festive seasons and, therefore, can make better inventory distribution.
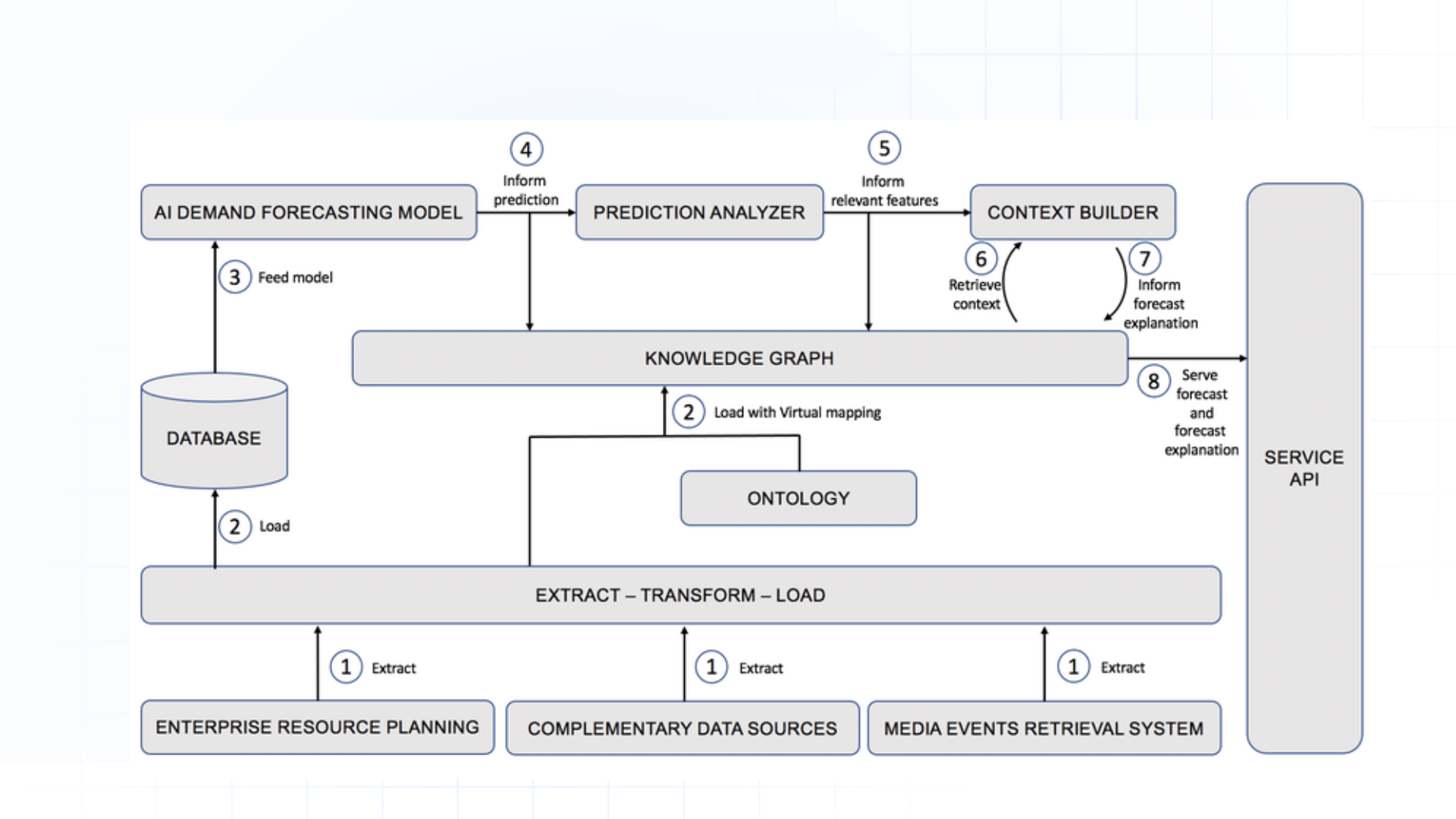
2. Inventory Optimization: Too much stock hinders capital utilisation, and if inventory is inadequate, companies lose sales. I have also found predictive analytics helpful in managing inventories properly since the system can suggest what is needed and when the time is right to restock, minimizing the issue of wasted stock.
Example: For instance, P&G uses forecasting models to tune the inventory and thus keep the necessary products in stock without overloading them.
3. Suppliers’ Performance Evaluation: Data on suppliers can be analyzed to foresee when there is likely a delay or a poor-quality supply, which can help deal with the supplier appropriately. A performance evaluation uses models to determine the effectiveness of criteria such as delivery reliability and defect rates to identify the best suppliers.
Example: One strategic application at Unilever uses predictive analytics to manage supplier risks and improve supply chain security.
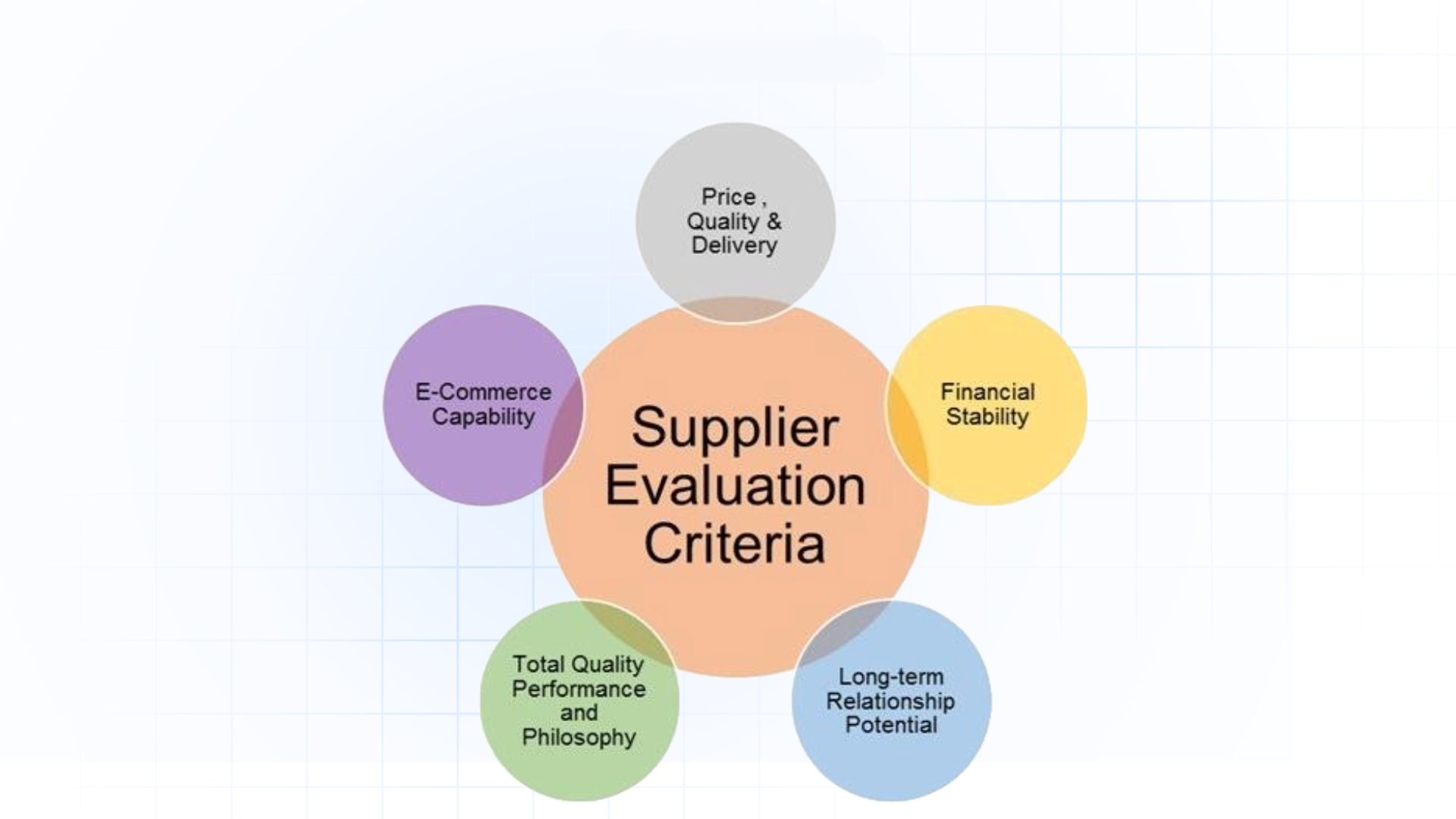
4. Optimization in Transport and Logistics: Transportation costs and even transport time losses are some of the significant challenges faced in supply chains. Predictive analytics models can use traffic records, weather conditions, and shipment history to determine delivery routes and timings.
Example: FedEx uses artificial intelligence and predictive analytics to deliver better customer value while reducing supply chain expenses.
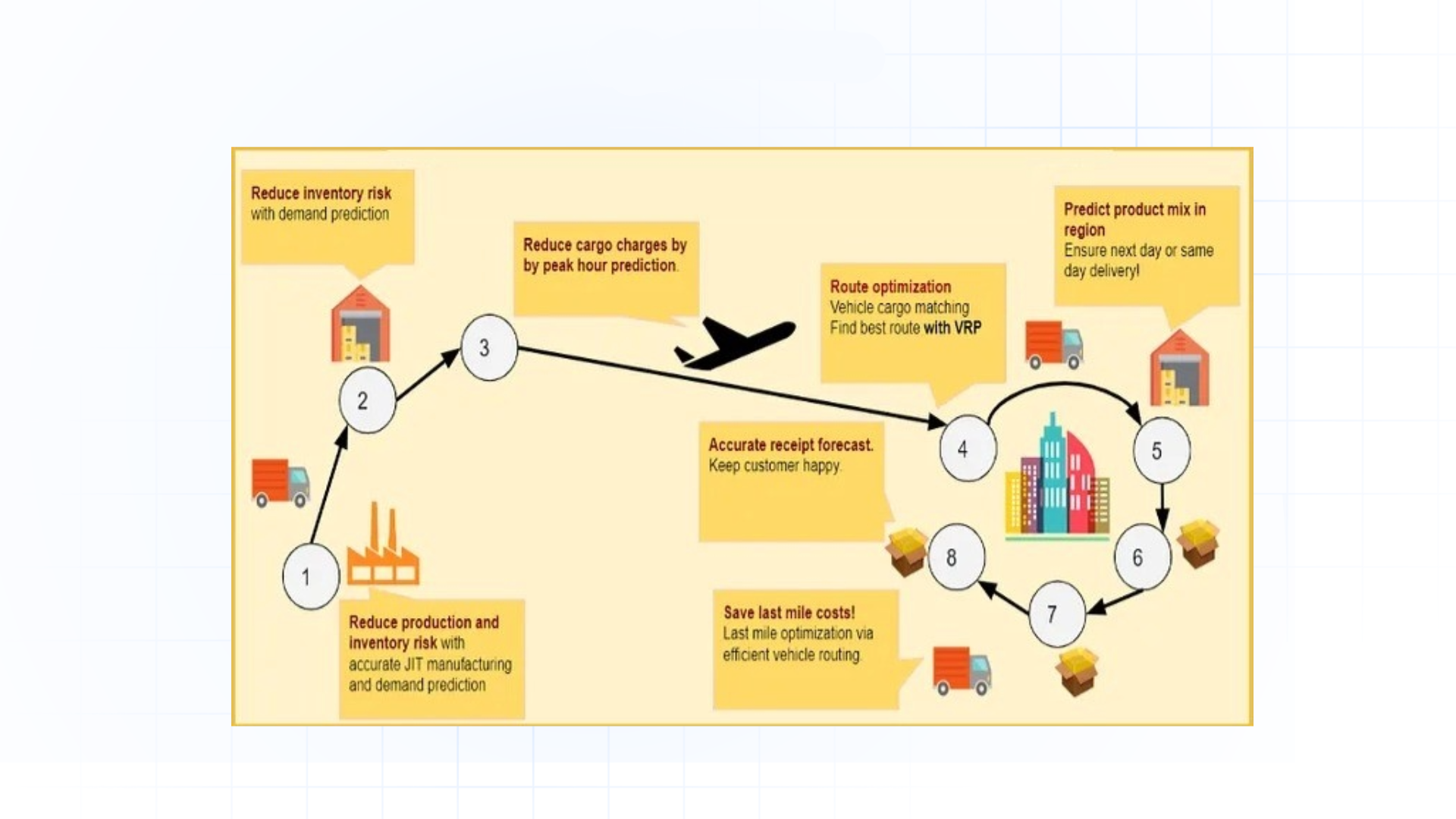
5. Risk Management and Disruption Management: Predictive analysis helps one realize what can go wrong globally, including port closures, strikes, or geopolitical scenarios. Companies can then devise countermeasures to reduce the effects.
Example: Coca-Cola has adopted predictive analytics to manage geopolitical risk and change the supply chain system.
6. Sustainability in Supply Chain Management: By suggesting efficient routes and customer orders, removing inefficiencies, and enhancing supply chain designs, predictive analytics assist companies in achieving long-lasting sustainability.
Example: Nestlé uses analytics to manage transport logistics differently, cutting down CO2 emissions and fueling requirements.
Transforming New Technologies to Supply Chain Forecasting
- Machine Learning and AI: Machine learning algorithms consistently improve because the system learns from new data inputs. AI also brings other new features, such as Natural Language Processing for News and Reports, to predict disruptions.
- Big Data Integration: Technical skills involve utilizing IoT sensor data, ERP system data, and even data from other sources, such as weather information, as inputs to increase the efficiency of the various prediction models.
- Cloud Computing: AWS, Azure, and Google Cloud are cloud planning tools for large-scale data generation and the running of predictive models.
- Digital Twins: Supply chain digital twins thus refer to stimulated models of the supply chains and can be used to test outcomes of decisions before having an actual occurrence.
- Edge Analytics: The implementation of edge computing proposes real-time predictive analytics by employing models below the layer near the data source, such as IoT sensors in manufacturing lines or delivery vehicles.
Current Trends
- Autonomous Supply Chains: Autonomous supply chains where an AI system makes decisions without human intervention are founded on predictive analytics.
- Integration of ESG Goals: Predictive analytics in supply chain management helps integrate business process locations with ESG goals to achieve high efficiency in sustainable and ethical processing accomplishments.
- Better Communication with Blockchain: The combination of PAM with the distributed ledger technology of blockchain improves supply chain credibility and shortens the time of revenue forecasting based on validated data.
- Multi-Scenario Forecasting: The systems have evolved today to do what experts call multi-scenario forecasting, whereby companies can develop several assumptions about how things might turn out under different conditions of either increased or decreased demand or supply shocks.
- AI-Powered Procurement: Sophisticated applications are designed to help recognize trends in commodity prices and supplier performance and advise on procurement strategies.
Barriers to the Use of Predictive Analytics
- Data Quality Issues Deficiency, error or inconsistency in the data collected negatively influences the efficiency of the decision-making models.
- Distribution across Enterprise Applications Combining predictive analytics with ERP or supply chain management systems is a big challenge for many organisations.
- High Initial Costs Each analytics phase involves investment in technology and infrastructure, skilled manpower, and other resources.
- Resistance to Change It has implications for organizations that they could struggle with employee rejection due to a lack of knowledge about AI ventures.
Solutions:
- Described approaches combined thus guarantee the quality of data collected through regular audits.
- This is because cloud-based analytical platforms make integration much more manageable.
- Team member training and a slow implementation process can reduce resistance and bring costs to reasonable levels.
Real-World Success Stories
Amazon: One of Amazon’s most important applications for big data is its ability to predict what the company’s stores need to stock and where. Its machine learning models help predict product trends to ensure it meets customers’ orders faster predictive analytics in big data.
PepsiCo: Retail Wooden’s big data applications include route optimization, which allows for the timely delivery of products to the respective stores at lower transportation costs and reduced carbon footprint.
IBM’s Watson Supply Chain: Watson of IBM targets supplying-chain administration and risk evaluation of supply batches in business processes by offering predictive analytics to businesses.
Future Outlook: The Path Ahead
At the centre of the above, ramming and predictive analytics are positioned to revolutionize supply chain management further with new technologies such as AI, IoT and data science. The following trends will shape the future:
- Real-Time Predictive Insights: As the IoT becomes more commonplace, supply chains will also have permissioned access to immediate data predictions and subsequent actions.
- Collaborative Ecosystems: Based on predictive analytics, suppliers, manufacturers, and distributors will collaborate to ensure a transparent supply chain.
- Hyper-Personalization: These predictive models shall only address micro-markets so that consumer goods are available in areas that only require them based on their demand frequencies.
- Proactive Resilience: Disrupted industries will prevent occurrences that cause disruptions to happen by implementing plans to address them before the problem arises.
Conclusion
Analytics for Demand Forecasting is revolutionizing the consumer goods industry supply chain because it helps businesses prepare and improve performance at every step. Applying AI, big data, and IoT in the supply chain can achieve a quadruple economic, social, and environmental bottom line.
With these tools, the prospects of predictive analytics changing supply chain management strategies are infinite, thus making it a crucial asset to organizations that desire to stand tall in the ever-increasing market competition. This makes this version extensive as it gives a clear view of what predictive analytics entails about the supply chain, its challenges, and its prospects.