AI agents are revolutionizing risk management in banking by enhancing accuracy, enabling real-time monitoring, and automating responses. These intelligent systems, powered by machine learning and predictive analytics, empower banks to manage risks more efficiently and proactively. With advanced data integration, fraud detection, and compliance monitoring, AI agents are transforming how financial institutions detect and mitigate risks. The future of risk management lies in the continued adoption of AI, enabling banks to remain agile and resilient in a rapidly changing financial landscape.
Considering the steep increase of information in the modern world that is characterized by big data, it has always been challenging for most banks to manage risks. Classic risk management techniques are gradually evolving through such elements of artificial intelligence as machine learning, big data, and other AI agents. Every one of these intelligent systems mines large troves of data to identify patterns that banks can use to counter risks effectively and quickly. In this blog, we will endeavor to describe what is happening in the realm of AI agents in risk management automation, focusing, therefore, on the beneficial shifts for financial institutions, as implementing these technologies is crucial in today’s challenging economic climate.
What is Risk Management Automation?
Risk management automation is the extension of applied artificial intelligence and machine learning to enhance the risk and operation of an organization. This appears to decrease the levels of dependence on manual work while increasing the productivity of risk management operations. It includes data mining, evaluation of the associated risks in the real-time mode, and support for estimation.
These technologies will help an organization learn about such risks as soon as they materialize and then proceed to find solutions to overcome the risk. They help businesses be more effective at what they do, be ready for changes in the financial environment, achieve compliance, and protect their assets.
Overview of Risk Management Automation in Banking
AI agents are autonomously working systems that rely on artificial intelligence. These agents can handle much information, create patterns of that information, and then make decisions, essentially emulating some of the actions of a human brain. Due to this characteristic, they can adapt to any domain, such as data processing, customer relations, and more, and perform process automation. In the banking sector, such agents are incorporated into the risk management automation systems, making detecting, evaluating, and controlling risks easier.
In the risk management context, the AI agents will capture and process risk data from various sources to build a rich risk portfolio for banks. For example, they may use current daily transaction frequencies to identify money fraud or use market trends when considering potential threats. Using predictive analysis, these agents create a roadmap for a bank to operate while avoiding risk factors and being compliant with governing laws and regulations while improving performance. They make processes easier for banks to handle and help the financial sector respond effectively to threats and expand the security layer rapidly.
Traditional vs. AI-Based Risk Management Automation
Feature | Traditional Risk Management | Agentic AI-Based Risk Management Automation |
Data Processing | Manual data collection and analysis | Automated real-time data processing |
Speed | Slower response times | Instantaneous risk detection and response |
Error Rate | Higher likelihood of human error | Reduced errors through machine learning |
Scalability | Difficult to scale with growing data | Easily scalable with AI technologies |
Predictive Capabilities | Limited predictive analytics | Advanced predictive models and simulations |
Cost Efficiency | Higher operational costs | Lower costs through automation |
Compliance Monitoring | Manual oversight required | Continuous compliance tracking via AI |
AI Agents Enhance Risk Management Automation
AI agents act as the backbone of risk management automation, providing several vital functionalities:
- Data Integration: This multi-agent system is effective enough in the search for and accumulation of the necessary data, including the results of transactions, market research, customer feedback, and external factors, including economic indicators. From this information, they develop a synthesized risk picture for the organization. This vision allows banks to assess their risk profile more comprehensively and, therefore, more effectively.
- Risk Assessment: To produce risk scores, these agents rely on performance calculations based on well-developed algorithms and machine learning. They establish what potential risks are so that the risk liability of an organization can be determined. It is efficient, and it only makes sense that organizations are prepared to answer risk in specific manners.
- Continuous Monitoring: Agentic AI has some capability for real-time monitoring. It monitors value drivers, KPIs, and other risk indicators on an ongoing basis to ensure any variation from the norm or escalation in risks is detected. If one takes a more proactive approach, then threats also emerge much earlier and help in improving organizational resilience.
- Decision Support: AI agents play a crucial role in supporting decision-makers by analyzing vast datasets and generating actionable insights. They provide detailed analyses that help stakeholders craft strategies for mitigating identified risks. This data-driven decision support empowers organizations to make informed choices that align with their risk appetite and business objectives.
- Automation of Responses: Such agents undertake action to reduce the extent of risk in case of risk emergence. The actions involve notifying the affected stakeholders, reporting the case, or rearranging some assets to meet certain standards. These responses may have to be automated to allow an organization to respond swiftly when there are threats to operations and impacts.
How Agentic AI Optimizes Risk Management Automation
Agentic AI is a widely known platform for improving risk management automation utilizing its agency AI models. Specifically, it improves numerous functions of risk management through the use of specialized AI agents.
- Risk Assessment Agents: These agents bring large amounts of information into the assessment of credit, market, and operative risks. Using sophisticated formulas, they develop specific risk descriptions and numerical risk values, which allow the banks to concentrate on their key concerns to the requisite extent. It helps banking institutions to locate their resources and determine the steps to take to avoid risks.
- Fraud Detection Agents: Agentic AI employs fraud detection agents that essentially read the transactions to provide their owner’s information about likely fraudulent events developed through ML. These ag learn and get more efficient as other fraud methods are employed. They minimize the number of losses and improve an organization’s credit with users thus increasing satisfaction.
- Compliance Monitoring Agents: These agents are important so that the banks can be compelled to observe the legal prerequisites that have been laid down. It integrates regulations and internal policies and informs the user immediately about breaches of compliance. Given that compliance monitoring agents help in making rapid decisions on probable problems, they prevent regulatory fines and loss of company reputation.
- Predictive Analytics Agents: These agents leverage historical data and other modeling methodologies to predict some of the risks that may exist in the future, given the current trends. Although known as ‘predictive’ analytic agents, they allow banks to consider various scenarios and their consequences for countermeasures against possible future events to deal with them more effectively before they happen.
- Incident Response Agents: Incident response agents automate the response process when risks or anomalies are detected. They inform other concerned departments and undertake pre-identified actions regarding threats and threats, making the process less cumbersome for banks to deal with threats. It enables action that eliminates possible negative effects on operations to be taken on time.
Use Cases of AI Agents in Risk Management Automation
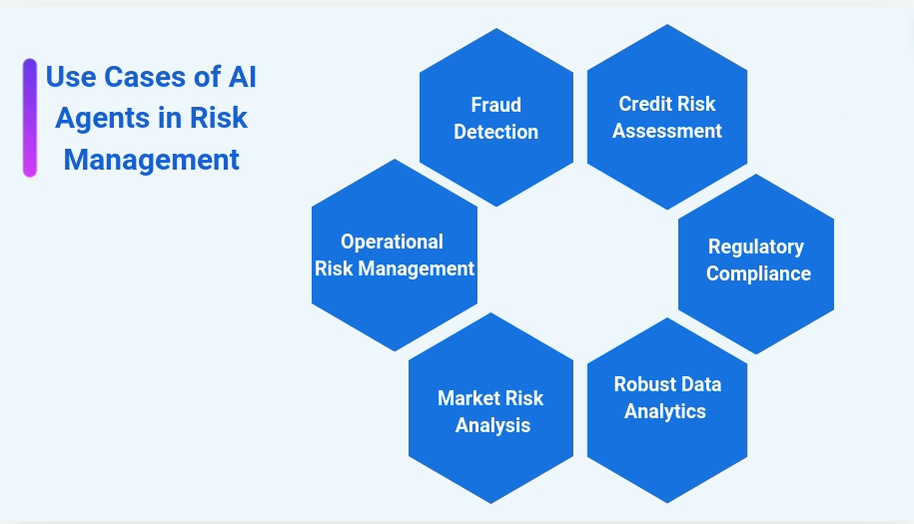
Applying AI agents in risk management automation yields numerous practical use cases across risk management.
- Fraud Detection: These agents can analyze transactional patterns and customer behaviors to identify potential fraud suspects. Usually, the transactions go unnoticed until they reach an alarming level, and therefore, banks can easily work on reducing losses before they escalate.
- Credit Risk Assessment: This multi-agent system evaluates borrower data, credit history, and market conditions to assess creditworthiness. It allows banks to make informed lending decisions while minimizing exposure to bad debts.
- Regulatory Compliance: Automated compliance monitoring agents keep update of the regulations and compare the bank’s business operations with regulations to ensure advanced compliance levels. They ensure that banks remain compliant, reducing the risk of fines and reputational damage.
- Market Risk Analysis: AI agents analyze the nature of the external marketing environment and how it might affect a bank’s portfolio. Banks can also change some of their investment plans in advance so that the consequences of fluctuations in the stock market can be escaped.
- Operational Risk Management: Agentic AI informs organizations about internal weaknesses, areas for improvement, and proposed changes, facilitating general functional stability and effectiveness.
Operational Benefits of AI in Risk Management Automation
The integration of AI agents into risk management processes brings several operational advantages:
- Enhanced Accuracy: With the help of algorithms and machine learning, agentic AI enhances the selectivity of risk evaluation. This makes it easier for banks to detect risks and greatly minimizes the number of manual mistakes. Sound risk profiles facilitate proper decision-making and optimized resource use.
- Real-Time Monitoring and Response: The AI agents make it possible to detect all the criminal hacks and risky factors in real-time, so the transactions are under constant observation. This proactive approach enables banks to respond quickly to potential issues. Swift action minimizes financial losses and operational disruptions.
- Operational Efficiency: Agentic AI frees up valuable human resources by automating repetitive tasks like data entry and compliance checks. Employees can focus on more strategic initiatives, increasing overall productivity. Streamlined processes enhance operational effectiveness across the organization.
- Proactive Risk Mitigation: Predictive analytics agents enable banks to anticipate potential risks based on historical and current data trends. This foresight allows for proactive measures to mitigate risks before they materialize. Enhanced resilience results from being prepared for emerging threats.
- Improved Compliance: Compliance monitoring agents help keep banks abreast of changes in legal standards. Notifications for the abovementioned deviations help to react quickly and minimize the probability of penalties. They also promote a firm compliance culture in an organization.
AI Agents Transforming Banking Risk Management Automation
Risk management automation in banking is being revolutionized through various AI agents and new trends in advanced technology. Here’s how:
- Machine Learning: AI agents leverage machine learning algorithms to analyze historical data and identify risk-related patterns. This technology enables banks to assess risks more accurately and develop predictive models forecasting potential risks.
- Natural Language Processing (NLP): NLP enables agents to solve news filtering text, regulatory changes, and social media feeds, among others. This capability assists banks in the external scanning of factors that affect risk, making them more aware of their surroundings.
- Real-Time Data Analytics: The agentic AI can make analysis of real-time data possible and help banks to notice new patterns and threats on the ground. For one, a rapid response capability is most valuable in fraud surveillance and operation risk mitigation.
- Robotic Process Automation (RPA): RPA integrated with AI agents automates repetitive tasks involved in risk management, such as data entry, compliance checks, and reporting. This reduces manual effort and minimizes the risk of human error.
- Predictive Analytics: Using predictive analytics, AI agents can forecast potential risks based on current and historical data trends. This forward-looking approach enables proactive risk mitigation strategies.
- Decision Support Systems: AI agents provide decision-makers with data-driven insights and recommendations. These systems analyze various risk scenarios and assist in evaluating the potential impact of different strategies, facilitating informed decision-making.
- Automated Compliance Monitoring: This multi-agent system monitors compliance with the requirements of the regulation by checking for compliance with established norms and generating signals for non-compliance. This way, the banks are kept in check, and there are fewer chances of having to face expensive penalties.
- Behavioral Analysis: AI agents can analyze customer behavior patterns to detect potential credit, fraud, and operational vulnerability risks. By understanding these behaviors, banks can implement more effective risk management strategies.
Future Trends in AI for Risk Management Automation
Looking ahead, several trends are likely to shape the future of AI agents in risk management automation:
- Enhanced Machine Learning Algorithms: As AI technologies advance, machine learning algorithms will become more sophisticated, enabling even more accurate risk predictions and assessments.
- Integration with Blockchain: Combining AI and blockchain technology can enhance transparency and traceability in risk management processes, improving data integrity and trust.
- Greater Personalization: AI agents will increasingly tailor risk management strategies to meet the specific needs of individual banks, considering their unique risk profiles and business objectives.
- Increased Regulatory Focus: As agentic AI adoption in risk management grows, regulatory bodies will likely develop new frameworks to ensure the responsible and ethical use of AI technologies.
- Collaborative AI Systems: Future AI systems may facilitate collaboration between banks, allowing them to share insights and improve overall industry risk management practices.
Conclusion: AI Agent in Risk Management Automation
The application of AI agents significantly improves risk management automation in the banking industry to ensure organizations have appropriate means of handling current and emerging risk situations. Banks can further improve risk management by realizing the benefits of the agentic approach of AI-based solutions over conventional methods and stand firm. Modern AI solutions optimize workflows and offer preventive measures against new problems to financial institutions. As the environment changes, their ability to adopt artificial intelligence solutions will be the key to ensuring that banks remain relevant, credible, and with the confidence of their consumers in the marketplace.